Investigators' Blog
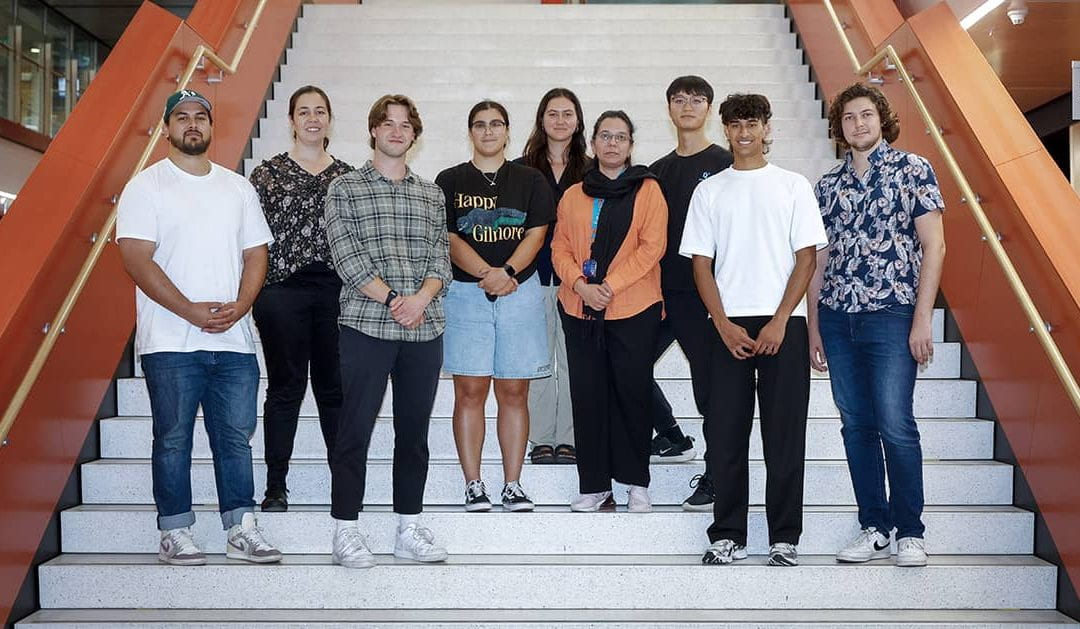
Internships support a new generation of researchers
Image: Te Pūnaha Matatini summer interns 2023-2024. Left to right: Jordan Phillips, Madeleine Barber-Wilson, Liam Gibson, Jaiya Bharti, Tiana Marshall, Zainab Rizvi, Jinglong Zhu, Mahin Panchia, Jared Clarke. Not pictured: Arwyn Whaanga, Halavaka Chapman, Tavake Tohi.
6 March 2023
A summer internship can be a lonely experience. That wasn’t the case for the 12 interns supported by Te Pūnaha Matatini over the 2023-24 summer.
As they worked on their diverse research projects in complex systems, the interns also came together to build whanaungatanga, experience the pioneering transdisciplinary approach of Te Pūnaha Matatini, and share their work with each other, supported by Te Pūnaha Matatini Deputy Director Associate Professor Mike O’Sullivan.
At Te Pūnaha Matatini we are growing and supporting a new generation of researchers who are ethical, collaborative, and do work that matters for Aotearoa New Zealand. This summer, our interns worked on projects ranging from the relationship between mātauranga Māori and science, how Pacific people perceive science in Aotearoa, enhancing an early warning system in a coronary care unit and improving virtual reality displays of a flood model, through to mathematically modelling lake health and investigating intergenerational language acquisition and transmission of te reo Māori.
“It gives us immense joy to see these interns coming through with all their skills and energy,” says Te Pūnaha Matatini Co-Director Associate Professor Priscilla Wehi. “It’s such a pleasure to interact and learn from them, and hopefully they will have learned something along the way as well.”
Madeleine Barber-Wilson (Ngāti Kahungunu ki te Wairoa, Ngāti Ruapani mai Waikaremoana) spent the summer working with Te Pūnaha Matatini Principal Investigators Associate Professor Graham Donovan and Dr Matiu Prebble as part of a project seed funded by Te Pūnaha Matatini to mathematically model the health of Wairewa Roto. Maddie explains that “shallow lakes are known to undergo abrupt transitions between a cloudy, polluted state with frequent algal blooms and a clear, healthy state based on changes in underlying conditions such as phosphorus levels. These critical transitions can be modelled mathematically, and our goal is to use a model to find mathematical early warning signals of changes in state for Wairewa Roto. This lake has particular significance as it is a customary eel fishery for Ngāi Tahu, so restoring its health means protecting a source of mātauranga and kai for iwi and hapū of the rohe.”
Te Pūnaha Matatini is the Aotearoa New Zealand Centre of Research Excellence for Complex Systems, with researchers and students based across the country. We coordinate an internship scheme for partner organisations including iwi, ministries, government departments, and private companies.
Mike explains that “we want to give our interns a small community to feel like they’re a part of over summer, because sometimes internships can be lonely. It’s really exciting to see such talented researchers coming through our education system, and the diverse topics that they are all contributing to.”
If you are from an organisation that is interested in collaborating with us on future internships, get in touch at tepunahamatatini@auckland.ac.nz.
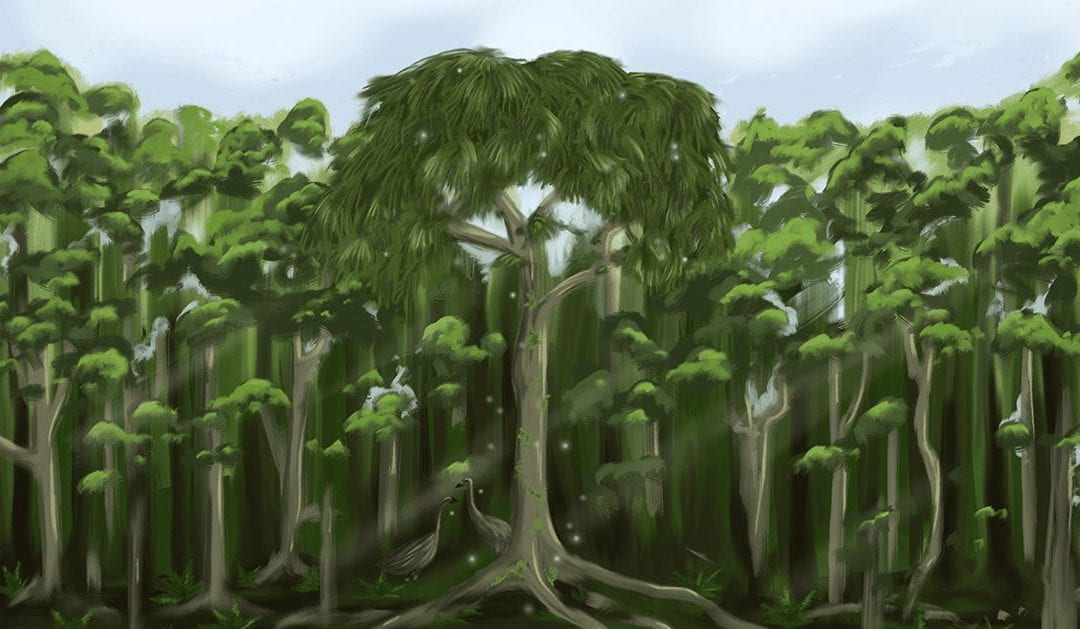
An ancient witness to changing land stewardship
4 March 2024
A collaboration between freshwater ecologist Kati Doehring and illustrator Jean Donaldson. Edited by Jonathan Burgess.
Tēnā koe – let me introduce myself. My name is dacrydium cupressinum, but most of you would know me as rimu. Gather close, for I have a story to share – a tale woven with resilience and hope.
My home lies nestled within a 400-hectare farm in the enchanting expanse of Dovedale Valley, cradled in the embrace of Te Tau Ihu, the top of the South Island of Aotearoa New Zealand. Here, amidst the ebb and flow of seasons, I have stood witness to the dance of life for centuries untold.
I have seen many creatures come and go. During my childhood, when my brothers and sisters were still standing tall next to me, big feathery chicken-like critters roamed my courtyard. Those were tranquil and harmonious days.
Then, during my teenage years, two-legged creatures appeared. Their needs were very different from mine, and they changed what used to be. It was in 1881 that the Win family became stewards of this land, altering the tapestry of my existence forever. John, the first generation farmer, did what was right at the time. They took my brothers and sisters, my aunties and uncles and used them to build their houses, churches and schools. They did what was right at the time.
I held on tight to what I had – my roots, my beliefs, my mana. I stood taller than ever at 60m in height. I am, after all, one of Aotearoa’s tallest forest species.
Life for me was in constant flux. Farming generations came and went – second, third and fourth – and with them the way they farmed. From Clydesdale horses to machines taking away my kin, then bulldozers root raking the soils that protected my veins. They put up fences to keep pigs, sheep and cattle in place, and secured hillsides and strengthened waterways to stop them from washing away. They did what was right at the time.
I watched how the land around me transformed into mosaics I had never seen before. Different creatures appeared, adding new colours to my courtyard. Goats, possums, magpies, wasps, old man’s beard and wild pigs spread like wildfire. And I stood tall.
Over the last two generations, I noticed more change and it made my roots tingle with excitement. Twenty years ago, the fifth-generation farmer, Arthur, together with his family, ensured that my family could come back and have a safe space to grow and thrive – two families bonded through time. They protected the patch of land I stand on so tall – all 25 hectares (61 acres) – and called it a Significant Natural Area. They did what was right at the time.
Today, under the stewardship of the sixth generation, led by Bryce, I am allowed to thrive once more. Together with his parents Arthur and Debbie, and Grandfather Bob, they farm for life, not just for a living. They do what is right. They reduced stock numbers over time and decreased fertiliser use on their farm.
As for me, I stand taller than ever. With each passing day, year, decade and century, I await the dawn of tomorrow with eager anticipation. I am an ancient witness to the many facets of land stewardship, observing the shift of environmental goalposts across the past and future of land management in Aotearoa.
Just the other day, I saw Bryce and his family plant a seedling, whose story can unfold under the protection of my canopy over centuries to come. They will do what is right.
Special thanks to Debbie Win for sharing the Win family story with us.
Kati is a freshwater ecologist who specialises in science communication, and a member of TPM Whānau. You can read more about her work with freshwater catchment communities here: https://ourlandandwater.nz/project/register-of-land-management-actions/
Jean Donaldson is a designer and native bird fanatic based in Te Whanganui-a-Tara. You can see more of her work at https://jeanmanudesign.com/.

International experts in complex systems gather in Aotearoa
Image: Dr Juniper Lovato presents at the Capital City Complex Systems Symposium 2024. Photo by Mark Coote.
23 February 2024
In a world facing unprecedented challenges, the need for innovative solutions has never been more pressing.
Last week Te Pūnaha Matatini brought together national and international experts in complex systems alongside practitioners in government, industry and community to explore how the study of complex systems holds the key to unlocking sustainable solutions for our most daunting challenges.
The Capital City Complex Systems Symposium 2024 interwove the latest advances in complex systems research with the concerns of communities, business and policy around how to ensure prosperous, resilient, equitable and just democratic life in a time of grand challenges.
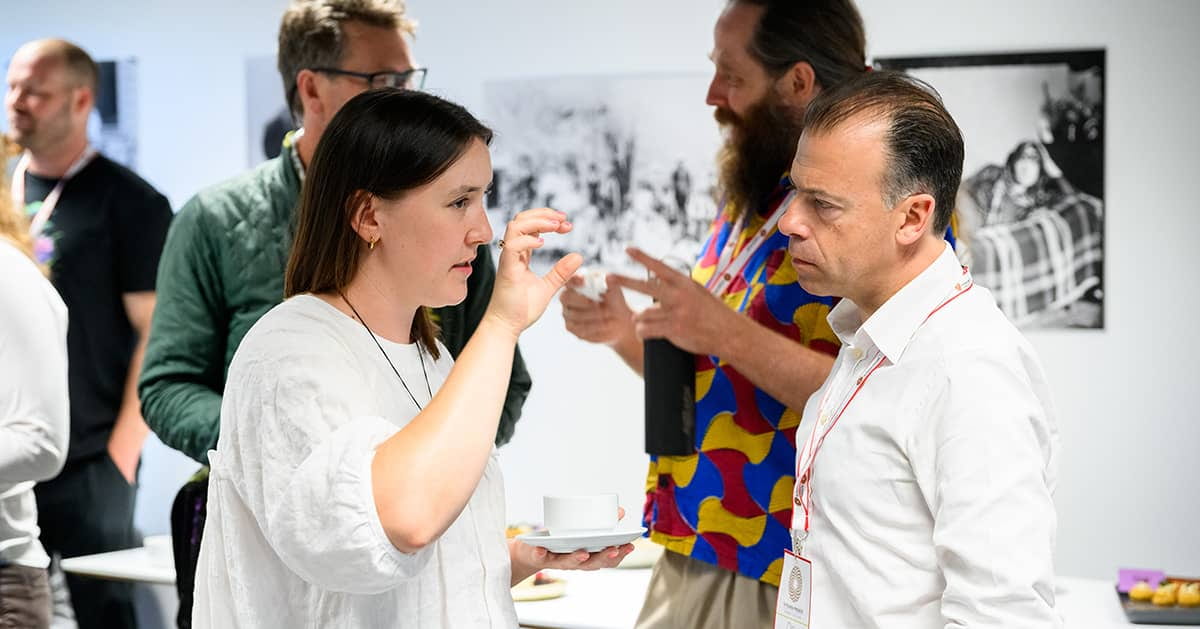
The Capital City Complex Systems Symposium was a chance to network with international complex systems experts.
Stephen Hawking famously said that the twenty-first century would be the “century of complexity”. Te Pūnaha Matatini has been leading the charge in Aotearoa New Zealand over the last decade with its pioneering transdisciplinary approach that applies complexity science to the critical and pressing issues of our time. “The issues we face – whether it’s building resilience against natural disasters, rethinking human mobility, preserving fragile ecosystems, or enhancing health and wellbeing – are deeply interconnected,” says Te Pūnaha Matatini Co-Director Associate Professor Priscilla Wehi. “They are not isolated problems with simple solutions but rather intricate puzzles demanding a holistic approach.”
The Capital City Complex Systems Symposium 2024 featured keynote presentations from complex systems experts from Vermont, Italy, South Korea and Australia. They spoke about issues of consent in socially networked environments, explored the complexity of human proximity networks, asked how we can unleash productivity through understanding creativity, and explored ethical dilemmas in disease interventions.

“The issues we face…are not isolated problems with simple solutions but rather intricate puzzles demanding a holistic approach.” Te Pūnaha Matatini Co-Directors Professor Markus Luczak-Roesch and Associate Professor Cilla Wehi. Photo by Mark Coote.
University of Auckland Deputy Vice-Chancellor, Research, Professor Frank Bloomfield, said that “the complexity of the world we live in has been brought to the forefront of our attention over the past few years. The University of Auckland is proud to host Te Pūnaha Matatini, a Centre of Research Excellence, and to be at the heart of the complex systems research community in Aotearoa New Zealand.”
Over two days, contributed talks challenged existing paradigms with innovative new approaches to biosecurity and disease transmission through to artificial intelligence, economic complexity and data science for transport policy. A stimulating poster session explored environmental policy reforms, the trajectory of te reo Māori language revitalisation, and creating simulation frameworks for entire hospitals.
Participants raced against the clock in lightning talks to explore the complexity of data in the public sector, data quality in large language models, the philosophy of complexity, and the relationships between research and policy. The symposium was purposefully single streamed with generous breaks, to build connections and community in complex systems research and practice.
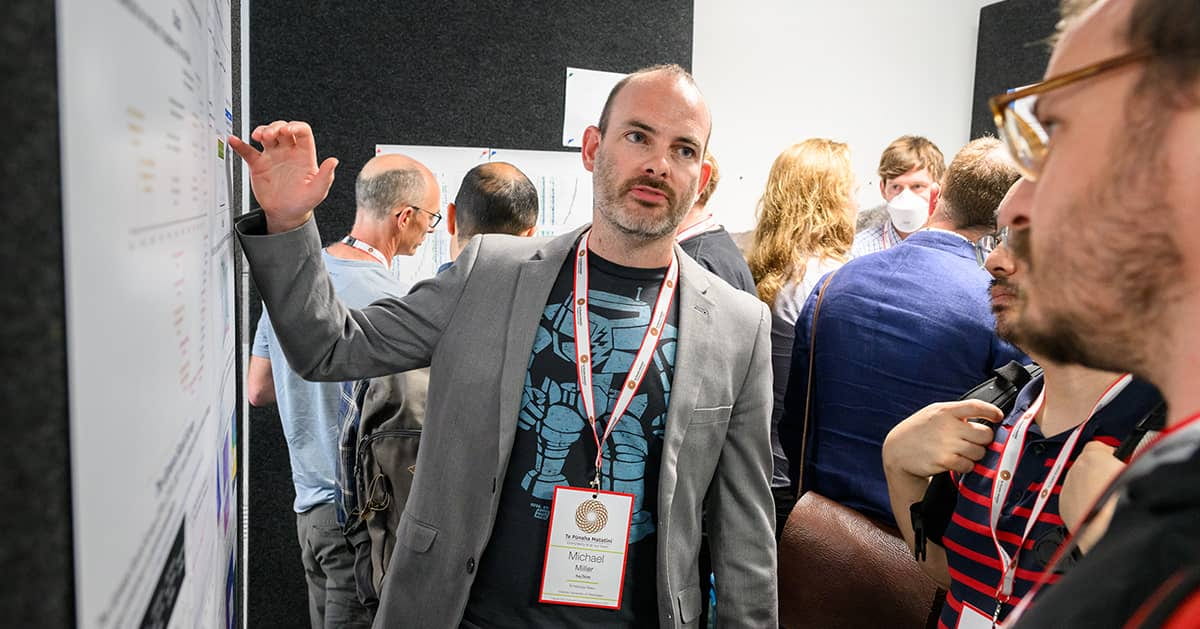
Michael Miller discusses the trajectory of te reo Māori in Aotearoa. Photo by Mark Coote.
Professor Nicola Gaston ended the symposium by making the case that complex systems researchers are uniquely well placed to understand matters of equity, inclusion, and diversity.
Representatives from government ministries and commissions, consultants, entrepreneurs and specialists in data science, sustainability and machine learning were among the participants. “This was a unique opportunity to learn from experts, network with peers, and discover new perspectives on complex systems to improve the lives of communities,” says Te Pūnaha Matatini Co-Director Professor Markus Luczak-Roesch.

The Capital City Complex Systems Symposium 2024 brought together 100 researchers and practitioners in complex systems. Photo by Mark Coote.
Te Pūnaha Matatini is the Aotearoa New Zealand Centre of Research Excellence for Complex Systems, and is hosted by the University of Auckland. It brings together 101 researchers in complex systems from across Aotearoa for collaborative and strategically focused research that creates significant knowledge transfer activities.
“Complex systems theory provides a groundbreaking framework that illuminates the hidden connections and feedback loops shaping our world,” says Markus. “By understanding the structure and dynamics of these systems, complex systems researchers provide invaluable insights into how small changes can ripple outward, creating transformative impacts on a global scale. It’s an incredible privilege that through Te Pūnaha Matatini we are part of the global network of leading scholars in this cutting-edge field of research.”
The next Capital City Complex Systems Symposium is planned for 2026.
Find out more about the Capital City Complex Systems Symposium 2024.
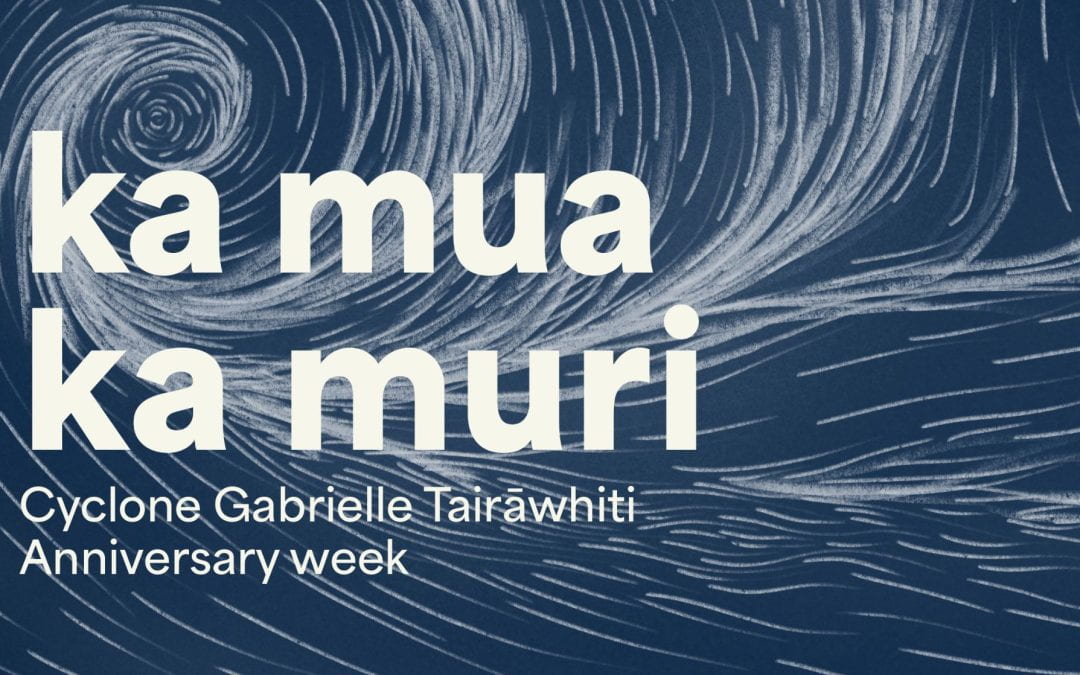
Hope and resilience in Tairāwhiti
31 January 2024
A week-long series of events from 9-16 February 2024 will mark the anniversary of Cyclone Gabrielle which devastated the East Coast of the North Island in February 2023.
In commemoration of the one-year anniversary of Cyclone Gabrielle’s impact on the Tairāwhiti region, Te Weu Tairāwhiti is organising a week-long series of events aimed at reflecting on the past, understanding the present, and envisioning a resilient future. Titled ‘Ka Mua Ka Muri’, the series of events will run from 9-16 February 2024 in communities around the region. Te Pūnaha Matatini is a co-sponsor of these events.
The main highlights of the week include a two day research symposium, a series of community hui showcasing local research undertaken over the past year, and a creative collaboration and exhibition showcasing regional climate and community initiatives.
Te Pūnaha Matatini Principal Investigators Professor Holly Thorpe, Professor Anna Brown and Associate Professor Mike O’Sullivan will be taking part in the events.
Anna is the head of Toi Āria: Design for Public Good, a research centre at Massey University. Toi Āria are collaborating with Te Weu to host a group of creatives from around Aotearoa to work with communities and projects around the region to have their experiences and perspectives interpreted through visual arts, music and creative writing.
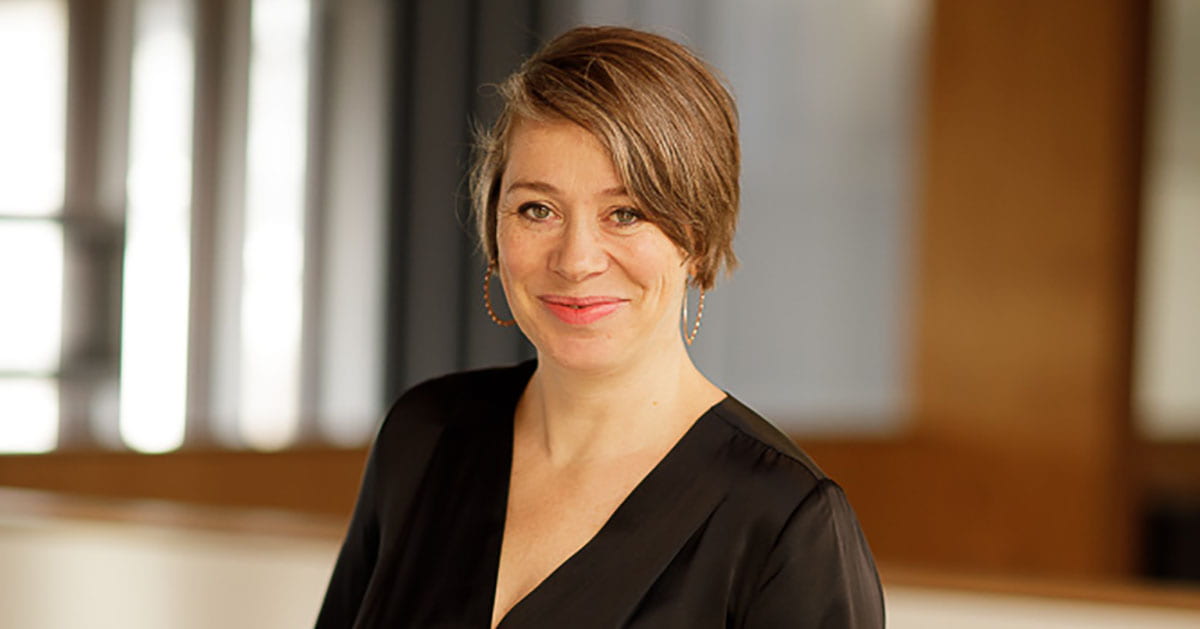
“We don’t know what will be produced but everyone is definitely looking forward to the process and outcomes!” – Professor Anna Brown
Anna, who has family in Tairāwhiti, says she was personally motivated to do something to support the region after seeing the impacts on her family and friends.
“When the cyclone hit and we were watching from afar we didn’t know how to contribute or help,” says Anna. “This project lets those of us from other areas contribute our creative skills and networks. We’re looking forward to getting alongside some of the amazing communities of Tairāwhiti to tell stories of hope and resilience. This project feels like a tangible way to support this region that still has a long way to go in the recovery journey.”
“We are bringing some of the country’s top creative talent including a jazz singer, a cartoonist, a multimedia designer, social media expert and a number of creative writers and journalists to jump into creative processes with Tairāwhiti’s artists and communities. We don’t know what will be produced but everyone is definitely looking forward to the process and outcomes!”
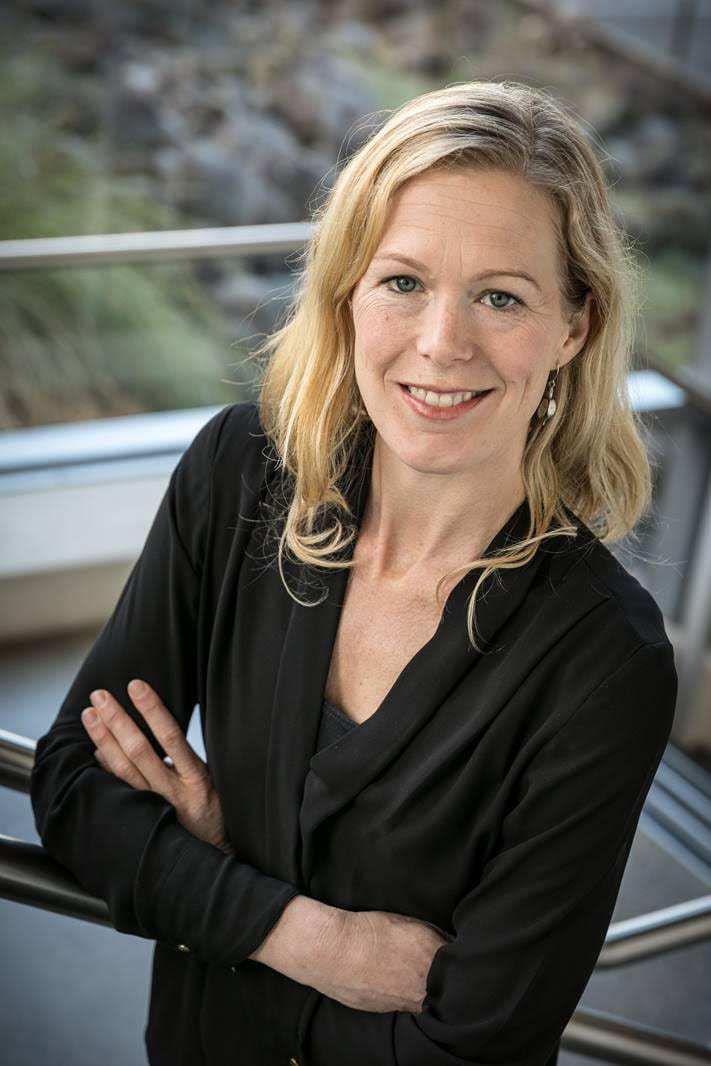
“These interviews from across the region are taonga that provide really important insights around what happened, what worked well and what could be done differently in the future.” – Professor Holly Thorpe
Holly has been involved in organising the research symposium, and will be presenting the work that she led with Josie McClutchie that explored the health and wellbeing impacts of extreme weather events in Tairāwhiti through interviews with nearly 50 health professionals and residents. “These interviews from across the region are taonga that provide really important insights around what happened, what worked well and what could be done differently in the future,” says Holly.
“It’s really important that the learnings this project generates are used locally, not just by central government. Repeated weather events have had a significant impact on the health and well-being of our communities. We also have some amazing stories of survival and support within neighbourhoods and villages. Health workers and services that were stretched throughout the Covid-19 pandemic have found ways to be innovative and look after the most vulnerable members of our community through this crisis as well.”
Mike O’Sullivan is deputy director of Te Pūnaha Matatini, and worked with Atawhai Tibble to form the Resilient and Sustainable Tairāwhiti (RASTR) group that is working with communities in Tairāwhiti. “RASTR connected with Te Weu Tairāwhiti early on,” says Mike. “We now have a bunch of new relationships and projects underway across the region from Anna Brown’s creative communities initiative as part of Ka Mua Ka Muri, to a distributed energy project, Holly Thorpe’s new project with rangatahi in the region, and work on digital ecosystems that can predict flood impacts within specific catchments.”

Wings, webs and wonders on the Chatham Islands
Image: Chrissie Painting and a student from Kaingaroa School learning about bugs.
26 January 2024
Written by Chrissie Painting and Tammy Steeves.
You can’t get much more remote than the Chatham Islands, a bumpy plane ride 840km east of Ōtautahi|Christchurch. Although the archipelago is made up of about ten islands, people live on just two: Rēkohu | Wharekauri | Chatham Island and Rangihaute | Rangiauria | Pitt Island. The rest are wild places, uninhabited by humans but important refuges for many threatened species.
A poignant issue for Chatham Islanders is that many miheke | taonga | treasured species were once found on Rēkohu and Rangihaute, but due to invasive predators are now restricted to these other islands that are tightly biosecurity-controlled and off-limits to the public. While visiting researchers are given the privilege to visit these remote islands, only a handful of residents have had the opportunity to interact with many of the species that make their islands unique.
With that challenge in mind, several Te Pūnaha Matatini investigators visited blustery Rēkohu and Rangihaute in October 2023 to connect with the community through a series of school visits, hui and a public outreach event. Chrissie Painting and Tammy Steeves, both principal investigators at Te Pūnaha Matatini, together with Roseanna Gamlen-Greene (TPM Whānau), Liz Parlato and Morgane Merien made up our team of five wāhine from five institutes, joined together by our shared values and passion for science. We were also joined by two special stowaways, Chrissie’s daughter (age 6) and Tammy’s son (age 10), who are both fabulous science communicators.
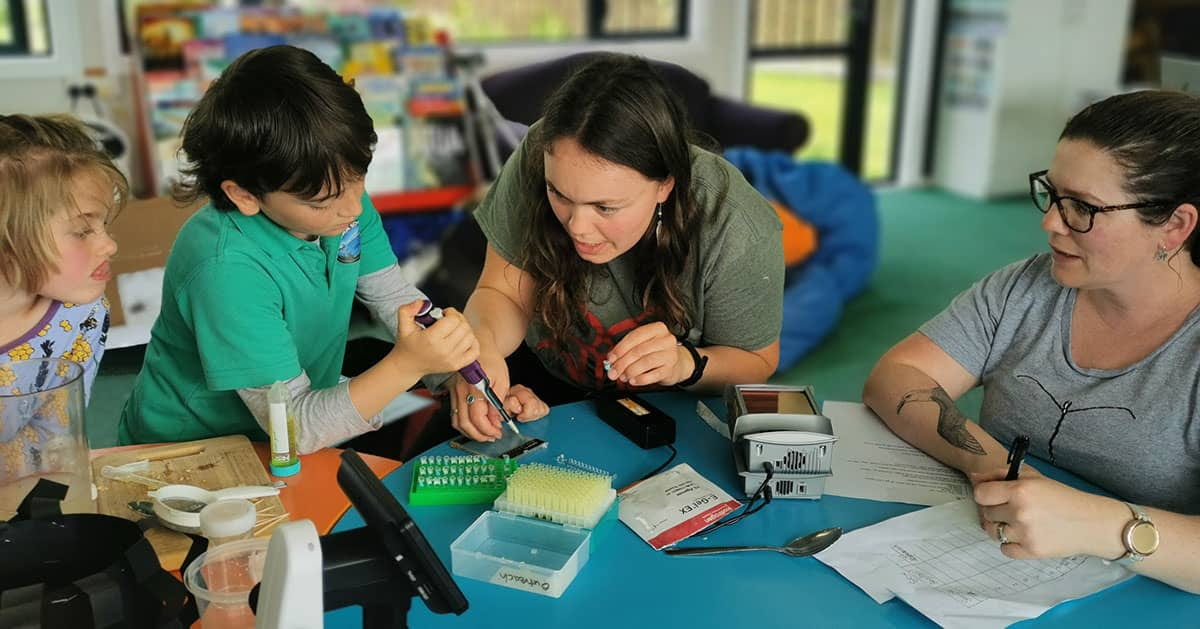
Roseanna Gamlen-Greene teaches students at Kaingaroa School how to pipette.
Our kaupapa was to facilitate connections: connections between scientists from mainland Aotearoa and the Chatham Island community, connections between Chatham Island people and endemic fauna, and connections between species (e.g. karure|kakaruia|Chatham Island black robin and their invertebrate prey).
One of those species is the Rangatira spider, a gentle giant famous for spooking late night visitors to the long drop on Hokorereoro|Rangatira island. It roams for wētā on tree trunks and is a relative to fishing spiders that have particularly whacky mating behaviour – which is what led Chrissie to propose her latest Marsden-funded project.
Despite a population size of only around 700 people, the Chatham Islands supports three schools: Kaingaroa, Te One and Pitt Island. However, the rolls are teeny tiny. At Kaingaroa, way up in the far north-west corner of Rēkohu, our own tamariki doubled the roll for the day, with just two kids in the whole school!
Just Tammy and Tate made it to Pitt Island this time around, where they explored DNA extraction and genetic sexing of birds with four fabulous students, and shared a few stories of how Tammy and her team use genetic and genomic data to help save threatened birds. The full team visited Kaingaroa and Te One, adding pitfall trapping to monitor backyard bugs and bug treasure hunts to the list of activities.
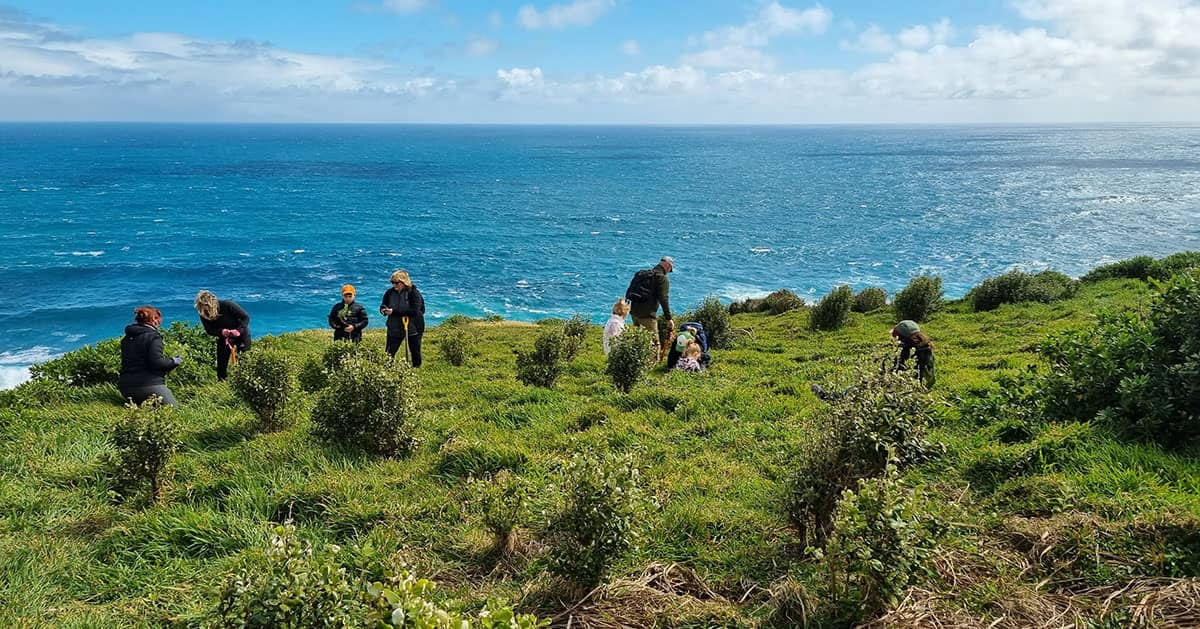
Planting trees at The Gap.
We also organised a weekend public event called ‘Wings, Webs and Wonders’, scheduled to overlap with the one day a week that Bubbles opens her café – it’s the only place and time you can get a serious coffee on the island! We set up a bunch of stands where people could inspect their pitfall trap catches under a microscope, paint a 3D Rangatira spider, and make a bowl of spider soup. We also had a series of genetic and bird-focussed interactive activities where people could extract DNA from split peas, genetically sex birds, eat like a black robin, learn to use pipettes and even touch one of the original traps used by Don Merton to rescue the last of the Chatham Island black robins from extinction. There was a great turnout, saved by the fact the final for the Rugby World Cup was the following morning and not at the same time as our event.
Our team had several special visits with imi | iwi. We had conversations about our favourite study species on Kōpinga Marae (Hokotehi Moriori Trust) over an amazing kai while our kids got to know the local tamariki by doing ninja rolls over mattresses in the wharenui. Tammy and Liz had a special tour of the work done by Maui Solomon (Moriori Imi Settlement Trust) and Susan Thorpe at Manukau, and Chrissie and Liz shared stories over a cuppa tea with members of Ngāti Mutunga o Wharekauri Trust.
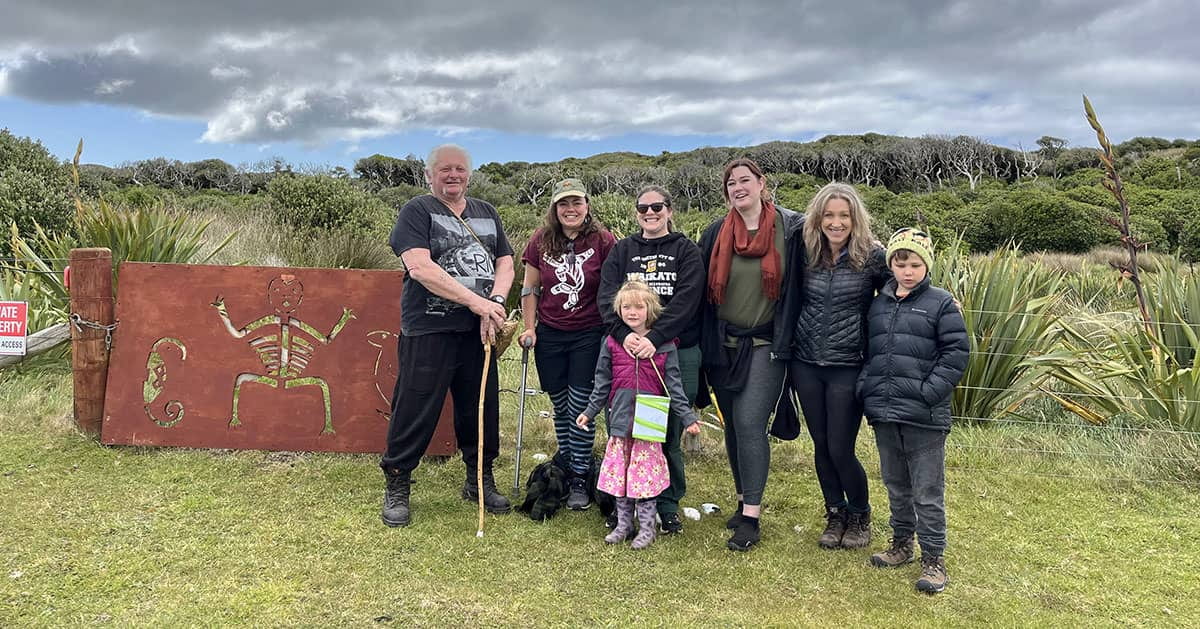
Visiting the kopi forest on Rotorua.
We were blown away by the hospitality of folks on the islands. Our team was treated to a visit to the rakau momori (sacred tree engravings) in the kopi forest at Rotorua, where we learnt moving stories of the Moriori people. It would also be remiss to leave out mention of the TWO occasions we had crayfish donated to us, where Tammy got to introduce the team to Canadian ‘lobster rolls’. Chrissie’s daughter got to boogie with the locals at the annual Halloween Disco, and we all felt rejuvenated by a visit to The Gap where we helped Department of Conservation rangers plant trees perched on the cliffs above the wild waves below.
We are all counting the days until we can return to this special place and its people.
The team were generously supported by seed funding from Te Pūnaha Matatini, the University of Canterbury Vision Mātauranga Development Fund, and two Marsden Fast-Starts (awarded to Chrissie Painting and Liz Parlato).

Wanting to ‘move on’ is natural – but women’s pandemic experiences can’t be lost ‘lockdown amnesia’
Header image: People wear masks on the street in Manhattan. Photo by Yoav Aziz on Unsplash.
15 January 2023
Holly Thorpe, Te Pūnaha Matatini, University of Waikato; Grace O’Leary, University of Waikato; Mihi Joy Nemani, University of Waikato, and Nida Ahmad, University of Waikato
The COVID-19 pandemic was – and continues to be – hugely disruptive and stressful for individuals, communities and countries. Yet many seem desperate to close the chapter entirely, almost as if it had never happened.
This desire to forget and move on – labelled “lockdown amnesia” by some – is understandable at one level. But it also risks missing the opportunity to learn from what happened.
And while various official enquiries and royal commissions have been established to examine the wider government responses (including in New Zealand), the experiences of ordinary people are equally important to understand.
As researchers interested in women and gender roles, we wanted to capture some of this. For the past three years, our research has focused on what happened to everyday women during this period of uncertainty and disruption – and what lessons might be learned.
Pandemic amnesia
Individual memory can become vague as time goes on. But this can also be affected by broader narratives (in the media or official responses) that overwrite our own recollections of the pandemic.
Political calls to “live with the virus”, and media hesitancy to publish COVID-related stories due to perceived audience fatigue, can create a collective sense of needing to “move on”. Looking back can be seen as questionable, or even attacked.
Indeed, misinformation and disinformation have been used, in the words of leading pandemic social scientist Deborah Lupton, to “challenge science and manufacture dissent against attempts to tackle [such] crises”.
But as the memory scholar Sydney Goggins has put it, such “public forgetting leads to a cascade of impacts on policy and social wellbeing”.
A gendered pandemic
Responding to the rapidly changing social, cultural and economic impacts of the pandemic, feminist scholars have highlighted the particular physical and emotional toll on women worldwide.
This has included social isolation and loneliness, increased domestic and emotional labour, the rise in domestic and gender-based violence, job losses and financial insecurity. Black, Indigenous, minority and migrant women have felt these impacts particularly keenly.
The same trends have been observed in Aotearoa New Zealand. And whereas some countries embraced pandemic recovery strategies that recognised these gender differences, this hasn’t been the case in New Zealand.
The gendered abuse of women leaders – former prime minister Jacinda Ardern and scientist Siouxsie Wiles, for example – have been well documented. But the experiences of ordinary women, their struggles and strategies to look after themselves and others, have had much less attention.
Experiences of everyday women
Our study involved 110 women in Aotearoa New Zealand. We set out to understand how they adapted their everyday practices – work, leisure, exercise, sport – to maintain or regain wellbeing, social connections and a sense of community.
Despite many differences between the women in our sample, there were also shared experiences. We referred to the ruptures in the patterns, rhythms and routines of their lives as “gender arrhythmia”.
The women responded to the psycho-social and physical challenges, such as disrupted sleep or weight changes, by creating counter-rhythms – taking up hobbies, exercising, changing diet.
The pandemic also prompted many to reflect on how their pre-pandemic routines and rhythms had caused various forms of “alienation”: from their own health and wellbeing, meaningful social connections, ethical and sustainable work practices, and pleasure.
The disruption of the pandemic caused many to reevaluate the importance of work in their lives. As one reflected:
COVID-19 has made me reassess what is the most important thing. Is it making money? Actually, no, not at all.
Others were prompted to question and challenge the gendered demands on women to “do everything” and “be everywhere” for everyone:
I think as women, because we’re so good at multitasking, we just put so much on our plates. I think we need to learn just to say no, because we’re not superhuman. And ultimately, all of this responsibility is weighing us down.
Our research also highlighted how the pandemic affected women’s relationships with familiar spaces and places. Leaving home for a walk, run or bike ride became important everyday practices that proved highly beneficial for most women’s subjective wellbeing.
Some came to appreciate physical activity for the general joys of movement and connection with people and places, rather than simply to achieve particular goals like fitness or weight loss.
Special challenges for young women
As part of our overall project, we also focused on 45 young women (aged 16 to 25). This highlighted the importance of recognising how gender, ethnicity and socioeconomic circumstances intersect.
Listening to their pandemic stories, we found young women played important roles in supporting their families and communities.
In particular, Māori, Pacific and others from diverse ethnic or migrant backgrounds carried increased responsibilities in the home, including childcare, cleaning, cooking and shopping. While many did so willingly, these extra burdens took a toll on their schooling, mental health and wellbeing.
For many young women, the pandemic was a radical disruption to their everyday lives and routines during a critical stage of identity development. They missed key milestones and events, and crucial phases of education and social development.
Many still grieve for some of those losses. And some are struggling to rebuild social connections, motivation and aspirations.
For example, some described being passionate and aspiring athletes before the pandemic. But social anxieties and body-image issues left over from lockdowns have been hard to shake, and have seen them struggle to return to sport.
The invisible work of migrant women
We also looked deeply at the experiences of 12 middle-class migrant women, and how prolonged border closures created real anxiety about “not being there” for families overseas.
As one nurse working on the front line of COVID care in NZ explained:
About a year ago, the cases of COVID in my homeland were increasing so rapidly. My family were not very well and I was depending on social media […] trying to reach out to them. I was really scared at that time, not being able to see your family when they really need you, not being able to be with them.
Some of the women in our sample also experienced increased anti-immigrant sentiments which further affected their health and wellbeing – and their feelings of belonging. As one said:
I’ve become extremely sensitive. I cry about small things. My doctor said “go and get some fresh air, it’s good for you” […] I went outside for a walk, and someone shouted at me, screamed at me. I got terrified for my life. How do you expect me to have wellbeing when no one in the society accepts you?
This arm of the research suggests a real need for investment in policies and support strategies specifically for migrant women and their communities in any future global health emergency.
Communities of care
A key feature of our study was the highly creative ways women cultivated “communities of care” during the pandemic. Even when they were struggling themselves, they reached out to friends and family – and particularly other women.
The majority of our participants were prompted to think differently about their own health and wellbeing, and what is important in their lives (now and in the future).
Throughout the pandemic, women have worked quietly, behind the scenes, in their families, communities and workplaces, supporting their own and others’ health and wellbeing. This invisible labour is rarely acknowledged or celebrated.
Many still feel the toll of economic hardship, violence and exhaustion. And less tangible feelings of disillusionment remain in a society that has so quickly “moved on” from the pandemic.
Acknowledging and addressing pandemic amnesia – personal and collective – is an important first step in documenting, learning from, and using these experiences to better prepare for future events. Next time, we need to ensure the necessary support is available for those most in need.
The authors wish to acknowledge the other members of the research team: Dr Nikki Barrett, Dr Julie Brice, Dr Allison Jeffrey and Dr Anoosh Soltani.
Holly Thorpe, Principal Investigator, Te Pūnaha Matatini, Professor in Sociology of Sport and Gender, University of Waikato; Grace O’Leary, Research Fellow, University of Waikato; Mihi Joy Nemani, Senior Lecturer, Te Huataki Waiora School of Health, University of Waikato, and Nida Ahmad, Research Fellow, University of Waikato
This article is republished from The Conversation under a Creative Commons license. Read the original article.

The past, the present and the future
20 December 2023
A collaboration between market analyst Hamza Ajmal and illustrator Jean Donaldson. Edited by Jonathan Burgess.
Objects are collections of essences.
I am sitting in my living room on a leather chair against a wall. The living room walls are off-white in colour with a few scratches, marks and scribbles on them. The chair is dark brown, made of leather and wood. The living room and the chair, according to the Ancient Greek philosopher Aristotle, possess unique essences derived from their materials and form. The walls are off-white, so they have an off-white essence. The chair is made of leather, foam and wood. It is formed by the amalgamation of these elements, creating the very essence of a chair.
The essences of an object have stories to tell.
According to Avicenna — the preeminent philosopher and physician of the Islamic Golden Age — the essences of an object are actually information. One can make informed inferences about an object by observing it. For example, the marks on the walls suggest movement of large objects through the living room that have hit the walls. The pencil scribbles suggest that children have played in the room. The chair has some chips and indentation in the seat, suggesting it is in a used condition, has been used for long sittings, and has been moved around.
The things that are old were once young.
Avicenna argued that when the chair was originally made, it stood untouched by the hands of time. As the days unfolded, however, information inscribed itself upon the chair — each scratch, movement, and sitting contributing to its evolving story. Each marking tells a story of the chair’s past and is not a mere imperfection. In other words, there is a relationship between information and time. As time goes by the universe increases the amount of information.
Avicenna — elaborating on this logic — argued that the world is not a collection of static things, but a dynamic flow of information across time. Instead of thinking about the future where there is more information, if we go back in time there is less and less information, and at some point, we reach a moment where there is the minimum amount of necessary information for an object to unravel itself from that moment.
The things that are soiled were once pristine.
The laptop I am currently using to write is three years old. It is well maintained, and has no signs of scratches or marks. I have taken good care of it, especially after my prior computer’s unfortunate encounter with Sprite and cappuccino. Fortunately, the laptop didn’t break. However, some of the liquid dried within the keyboard. For someone who likes Macbooks for their keyboard, typing with sticky keys is a pretty awful experience.
The markings on an object are results of actions.
Over the last few years, my newsfeed has become more and more rife with climate catastrophes, the endangerment and extinction of species, and potential threats to human survival. A part of my work is to observe and forecast external factors that can have an impact on New Zealand and the world economy. The climate pattern El Niño is appearing increasingly frequently amongst these factors.
Among the various risks associated with El Niño, global food security is a significant concern. For New Zealand, repercussions of extreme weather events are multifold, ranging from economic disruption to loss of life. A recent manifestation was Cyclone Gabriele in early 2023, the aftermaths of which are still lingering across the country and will continue to do so for some time. If we view the environment as the walls of my living room, the current state of my living room is a direct result of past actions.
Kia whakatōmuri te haere whakamua.
In Māori philosophy the conceptualisation of time does not leave the past behind; one carries one’s past into the future. These concepts are similar to the philosophical narratives of Aristotle and Avicenna that things are connected with time. If we seek to put things back in order, maybe it is a wise idea to revisit the past — where there are less and less markings on our environment — and learn from the practices of our ancestors that lived in harmony and connection with their environment.
I walk backwards into the future with my eyes fixed on my past.
Hamza Ajmal is a principal investigator with Te Pūnaha Matatini who researches in the areas of finance, economics, and sustainability.
Jean Donaldson is a designer and illustrator who works with Toi Āria: Design for Public Good. She is based in Te Whanganui-a-Tara. You can see more of her work at https://jeanmanudesign.com/.
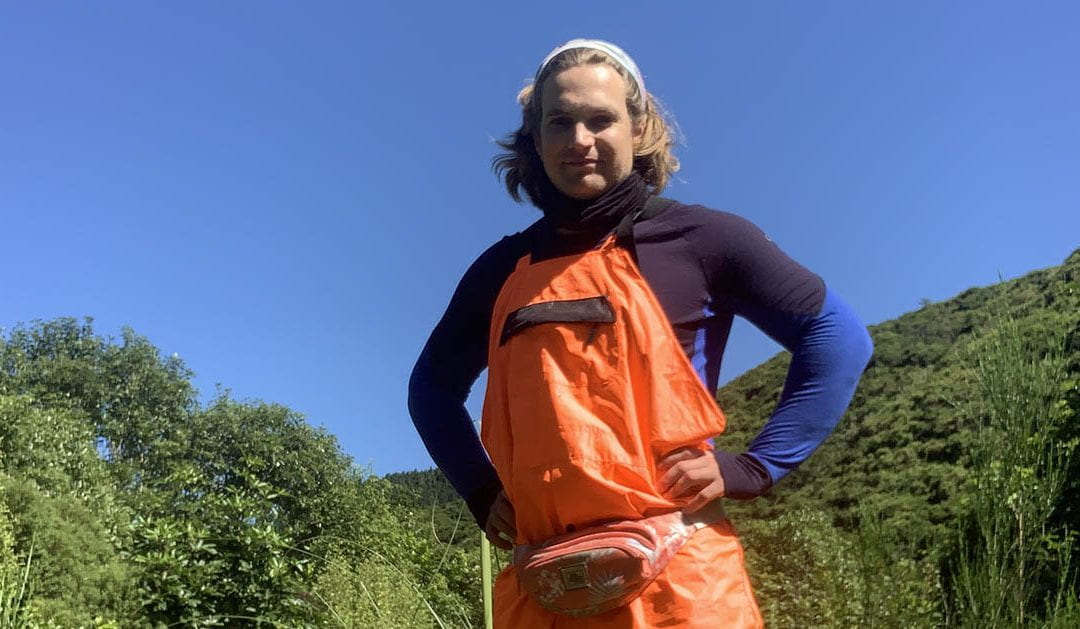
How can we ensure landscape-scale ecological restoration outcomes over time?
7 December 2023
After his recent fieldwork, Henry Morse had almost 9,000 photos of plants on his phone.
Henry has been collecting data on how ecological restoration sites have been regenerating over time, to contribute to his PhD in landscape ecology and ecological restoration. He recently received seed funding from Te Pūnaha Matatini to employ two research assistants to assist with his fieldwork.
Ecological restoration aims to recreate, initiate or accelerate the recovery of an ecosystem that has been disturbed. The sites that Henry has been researching are diverse. They are of different ages, different sizes and different locations. Some are surrounded by urban landscapes, some by suburbs, and others by farmland.
Along with his research assistants Paul Bell-Butler and Faustine Cabanne, Henry completed vegetation surveys at sites across the lower North Island of Aotearoa New Zealand, using transects. Transects are straight lines that cut through a landscape so that standardised measurements can be made, tracked over time, and compared to other areas. This will give him a full census of the seedlings, saplings and older trees at each site, and allow him to assess whether regeneration is processing well or being stunted.
Each site took around a day to survey, and half a day afterwards to process all the data — logging huge numbers of plant identifications and measurements, and turning those thousands of photos into usable data.
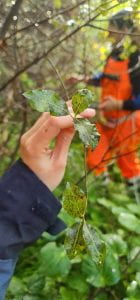
Image: One of the thousands of plant identification photos taken by Henry and his research assistants.
At the moment, ecological restoration in Aotearoa is largely driven by motivated individuals. “There are a lot of individual landholders that are interested in ecological restoration,” says Henry. “Some have land that is too steep for crops, or so boggy that they lose stock in it. Restoring degrading hillslopes can prevent further loss of that area, and restoring a bit of wetland on a farm can save the cost of having to pay for losing stock each year.”
“Other people simply have a passion for restoration.”
For ecological restoration to have real benefit, it needs to move beyond these farm-scale projects run by individuals to broader landscape-scale projects. Henry’s overall PhD project is looking at what species to plant, where to plant, and why to plant for landscape-scale restoration. In the first chapter of his thesis, he looks at species distribution models under climate change futures, to get an idea if what we’re planting in restoration projects makes sense for the long term.
The data from this fieldwork will inform the second chapter on how restoration sites are recovering, based on their place in the landscape. “Regeneration sites within a certain proximinity of a seed source might show good diversity and abundance,” explains Henry. “So they might need less effort than another site.”
This analysis will be used to strategise restoration on a landscape-scale. Planting is labour-intensive and expensive, so this research will be useful to prioritise where to plant, and what can be left to regenerate on its own. “Natural regeneration is probably going to be the primary powerhouse behind getting regeneration going on the landscape scale,” says Henry.
In the final chapter of his thesis, Henry will explore different landscape-scale restoration scenarios. “If we were to restore five metres alongside every major waterway, what would the impact on the ecosystem be?” asks Henry.
Henry is originally from Los Angeles. Once he has completed his PhD, he wants to continue working in the Aotearoa research system. “The ecological research that’s happening here is amazing,” he says.
“The cutting edge of where ecology is going now is being led by mātauranga Māori. Even though it’s not what I’m working on, just being in the proximity of that feels awesome.”
And if there is a restoration site or group near you, Henry encourages you to get involved. “They can really use the support, and it does so much for your mental health.”
“Or just simply walk off the trail,” says Henry. “Wander around a little bit and get a feel for your local ngahere. It’s awesome.”
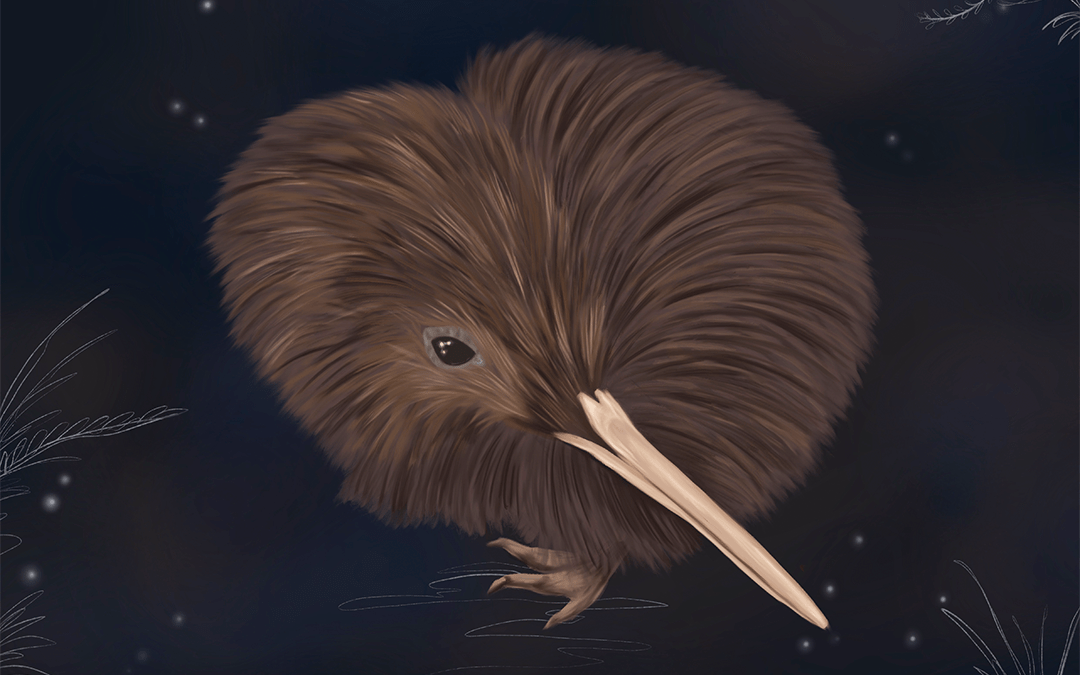
The night time world of the kiwi
4 December 2023
A collaboration between wildlife biologist Isabel Castro and illustrator Jean Donaldson. Edited by Jonathan Burgess.
Kiwi are a group of five extant species of birds endemic to Aotearoa New Zealand. Their bodies are rounded, and they have a long bill that curves down at the end of a long neck. They belong to an ancient group of birds at the base of the avian tree of life. They have tiny wings that are useless for all intents and purposes, but a very large brain for a bird. Their brain is large due to the enlargement of several of the areas needed for cognition (innovative feeding, social behaviour, and vocal learning) and integration of information.
Kiwi are nocturnal. They are active during the dark hours and rest in burrows or hideaways during the daytime. Being nocturnal poses many challenges. They have very small eyes, but a highly-developed sense of smell — something that can be used in the dark to get information about their surroundings, especially other animals.
I always encourage my students working with kiwi to try to become a kiwi by sitting in the darkness of a moonless night on the forest floor. I ask them to close their eyes and take some time to adjust to the darkness, inside and out. We must close our eyes as our sense of sight is so strong that having them open would interfere with other sensations.
The sense of touch becomes important when one cannot see. Feeling the air and the cold around bare patches of skin — especially the face and hands — can help with navigation, social interactions, and gathering weather information. By listening to the sounds around us we can identify animals moving in the understory, mosquitoes approaching, and the song of the nocturnal species like ruru allows us to know about other kiwi, predators, and parasites.
I ask them to smell the air, and ask what is in it. What plants can they recognise? Animals? What can they say about their environment as they use their senses like kiwi? When they get up, can they walk safely? What would they need to walk?
After a few tries, a person can distinguish between the noises made by rats, mice, donkeys, sheep, kiwi, other birds roosting in the trees, insects, and other humans when walking in the bush. They can also detect the presence of streams by their sounds and tell the height of the canopy by listening to the wind on the tops of trees. Smelling is not as easy, but we can easily smell the flowering plants in an area, wet soil, and many smells brought in by the wind — many we cannot identify. We can walk aided by a stick or by placing our arms outstretched. The easiest is going on all fours!
Although not confirmed, I imagine that kiwi use their large brain to form mental maps of what is in their environment. I think it could be like having a map or GPS that allows them to move safely around as long as things stay in the same place. In case something drops on to the forest floor, or moves, they use their tap-ahead behaviour, where they touch the ground in front with their bill as they move, to make sure they do not trip on things. In addition to using air movement around their body, sounds and smells, this would allow kiwi to have a pretty good picture of what their area is like.
By touching obstacles with their bill, kiwi can also update their mental maps by adding new obstacles. When a tree or a new plant falls on the forest floor, many kiwi in an area come to it and for the first few days of falling they have a heightened interest in it. I think this allows them to add it to their map.
When we were studying the foraging behaviour of kiwi my PhD student Susan Cunningham and I would find a kiwi in the darkness of the night while walking around with a low light head torch and would stop to video him or her using infrared technology.
We recognised the kiwi because of two main bits of information they provide while out at night. Firstly, they looked like a dark basketball (their body) with a bright white stick (their bill) in front of the ball. The reason why the bill is so prominent in the dark is unknown, but it could be possible that it reflects light in a special way that makes it more visible. That way kiwi could find each other by looking at the whiteness of each other’s bills.
The other giveaway is their extremely noisy movements — especially in the middle of a dry spell when the understory is crunchy underfoot. Kiwi are so noisy one could think it is a human walking around!
Once we stopped, we would start to video their behaviour. Invariably, the bird would be at least ten metres away from us, but as time went by they would get closer and closer while seemingly indifferently smelling in our direction. We took it that they were interested in us and our presence and approached to check us at a range that would allow a better understanding of what we were. If we moved and made a sound or coughed or cleared our throats, they would run a short distance away and start the process again.
While kiwi can call and they have different sounding calls for males and females (and possibly individuals), they only call on average about once per night. We found that pairs and groups that nest together tend to be within earshot of each other’s movements and I wonder whether these close-range sounds are used to know the position of the members of their social group.
I imagine that this would be like recognising the walking pattern of a familiar person. It would be super interesting to record such sounds and see whether individuals have specific identifiable patterns.
Isabel Castro is a principal investigator with Te Pūnaha Matatini who researches how an animal’s biology and behaviour affect its conservation status.
Jean Donaldson is a designer and illustrator who works with Toi Āria: Design for Public Good. She is based in Te Whanganui-a-Tara. You can see more of her work at https://jeanmanudesign.com/.
Special thanks to Professor Stephen Marsland, who co-leads the Ponui Island Kiwi Research Programme, David Chamberlin and family who own our study site and are members of our team, and the many postgraduate students who help us discover amazing facts about kiwi.
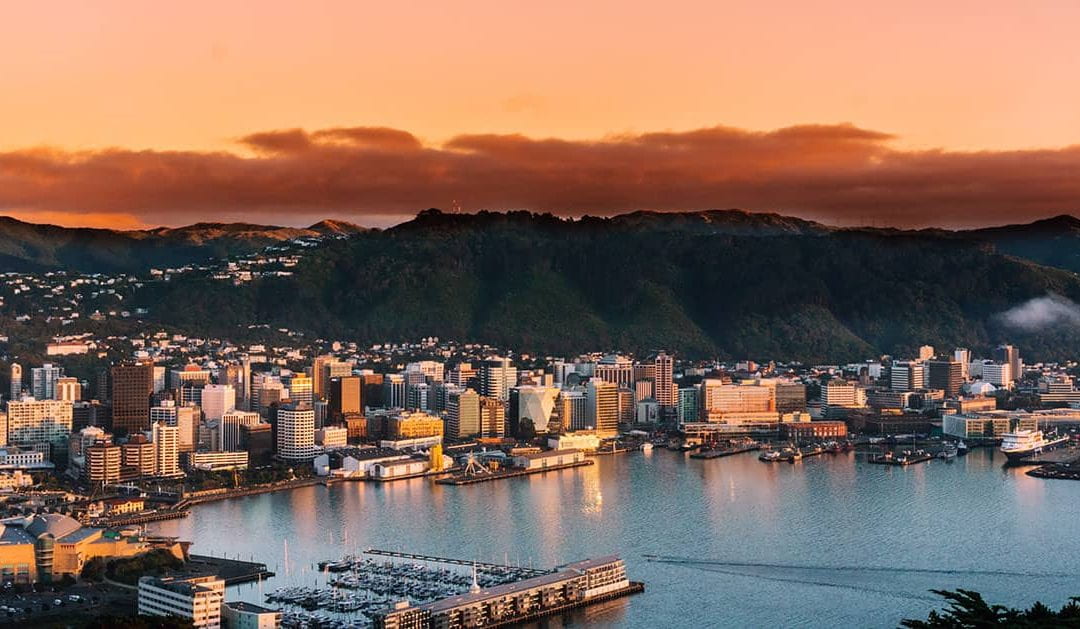
Capital City Complex Systems Symposium 2024: Call for abstracts
23 November 2023
The call for abstracts for the Capital City Complex Systems Symposium 2024 has been extended to Friday 1 December 2023.
Thank you to everyone who has registered for the Capital City Complex Systems Symposium 2024. We’re really looking forward to seeing you in Te Whanganui-a-Tara in February to kōrero all things complex systems, share knowledge, and build new collaborations.
We have lined up an exciting list of international keynote speakers and are now inviting abstract submissions for contributed talks, lightning talks, and posters.
Talks will be given 15 minute slots (12 minutes presentation + Q&A). Poster presentations will also include a short mini-presentation (no Q&A) to advertise the poster, followed by a poster reception to facilitate in-depth discussions with symposium attendees.
If you would like to present at the symposium, we’re asking for a short abstract (max 250 words) on complex systems, network science, and their applications by the 24th of November.
Abstracts will be reviewed by the programme committee for rigour, significance and suitability, with a focus on balancing the programme diversity with thematic groupings and synergies.
Submission deadline (extended): Friday 1 December 2023
Notification of acceptance: Friday 15 December 2023
Submit an abstract for the Capital City Complex Systems Symposium 2024 – Google Forms