Guest Blog

Countdown – an extract from Shaun Hendy’s new book, The Covid Response
16 June 2025
An excerpt from Shaun Hendy’s new book, The Covid Response – A Scientist’s Account of New Zealand’s Pandemic and What Comes Next.
Just after 1.48 p.m. on Monday 23 March 2020, Prime Minister Jacinda Ardern announced that, following the recently introduced four-level alert system, New Zealand would move to Alert Level 3 (‘Restrict’) and then Alert Level 4 (‘Eliminate’) in the next forty-eight hours. Schools and most businesses would close, and travel would become severely restricted. Ardern had introduced the system just a few days earlier and already calls for her to make use of it had been growing. For some people her call came too late. For others it was a grave mistake. It was unprecedented. It was surreal.
I watched the announcement live, but when I think of that day I relive a moment from earlier in the morning. Just after 8 a.m. I was alone, sitting in my car in the driveway, in a cold sweat. My wife was still inside, gathering her coat and bag, on her way out to join me. I was now working from home, but she was still required to go to her office in Auckland’s CBD. I’d offered to drive her so she could avoid her normal commute on a crowded bus.
I was still sweating from a live interview with John Campbell from Television New Zealand (TVNZ). It was the first time I had appeared on TV via a video-call. During the interview I sat at my computer, newly set up on our dining table, and tried hard to keep my eyes on the bright green LED that marked the location of the webcam.
Just a fortnight ago, I had pulled together a team of scientists from around the country to model the spread of a new coronavirus. As a physicist it was not something I had imagined ever having to do, but I had worked with the government on animal diseases before and had found myself fielding requests from officials as the coronavirus got closer. Now I was in demand from the media.
I had plenty of experience with live TV, but I’d never given an interview like this before. Excerpts from it still play in my head today. I told Campbell that it was likely several thousand New Zealanders would die as a result of the novel coronavirus. If we did nothing, I said, the number of fatalities might be in the tens of thousands. Sharing these possibilities with Campbell’s audience was one of the most terrifying five minutes of my life.
Sitting on my own in the car, I thumped the steering wheel as I questioned what I had said. Did I get the emphasis right? Should I have been more optimistic?
Some scientists, like seismologists and volcanologists, prepare for such a moment for decades, studying the best ways to communicate in an emergency by reading academic articles, reviewing how their predecessors performed in the last disaster. The challenge is similar to communicating about a severe weather forecast. If you focus on the best-case scenario, people won’t take it seriously, but if you overemphasise the worst case, some may overreact. As a physicist, I had more experience in debunking claims about mobile phones causing cancer than grappling with how to deliver the kind of bad news that pandemics create. I was shaken.
I took a deep breath to clear my mind, picturing the route I would take from Grey Lynn to the CBD. My wife joined me in the car, and I backed slowly out of our driveway, my eyes on the rear-view mirror. It was a relief to be able to focus on driving.
I could hear an edge in my voice, though, as we talked about what might happen later that day. ‘The Prime Minister is likely to send everyone home today,’ I said. I offered to drive into town again to pick her up after the announcement, along with anything she needed from the office to work from home for a few months. ‘There could be a rush, so I’ll try to leave home early, maybe even before the press conference is over,’ I told her.
Traffic into the city wasn’t bad, halving what would normally have been an hour-long round trip. Perhaps people were avoiding the CBD. Just that morning, New Zealanders had woken to the news that the death toll from the new coronavirus had risen to 281 in the United Kingdom, while epidemiologist Sir David Skegg had told Radio New Zealand that we were just eight days behind. Mike Hosking, Newstalk ZB’s popular breakfast host, told his listeners: ‘You add cases by ones, then handfuls, then you add them by [tens], then you add them by hundreds.’
I ate lunch in what had been my kitchen but was now my office, listening to Dr Ashley Bloomfield on the radio. New Zealand had thirty-six new cases that day, bringing the total number of confirmed cases on our shores to 102. At around 1.30 p.m. I switched on the TV for the post-Cabinet press conference. A few minutes later, Prime Minister Ardern stepped up to a lectern in the Beehive.
Fifteen minutes into the press conference, Ardern told New Zealand that we would move to Alert Level 3 immediately and then Alert Level 4 at the end of the day, on Wednesday 22 March, for four weeks. Just a few weeks earlier such a decision would have been unthinkable, to me and to many other people, but as I watched Ardern lay out her plan, it now seemed an inevitability. What other choice did we have?
Two days later, my colleagues and I met by Zoom at 9.30 a.m. to discuss work priorities for the day, talk about any problems we were encountering, and set up any other meetings we would need later in the day. This routine would anchor my work life for the next two years. During this early period, in late March and early April, we stuck to it seven days a week.
At 12.21 p.m. that afternoon, a National State of Emergency was declared. The State of Emergency gave officials extra powers, allowing the government to mount a quick response to developing events. This was initially intended to last until 12.21 p.m. on 25 April, but it was extended six times, finally coming to an end on 13 May. It would be the longest state of emergency in New Zealand since the Canterbury earthquakes in 2011.
Then at 11.59 p.m., New Zealand moved to Alert Level 4: Eliminate.
The Covid Response (BWB, $39.99) is available now from all good bookstores.

A new approach to tackling gender pay gaps in New Zealand’s public sector
13 June 2025
A new study from researchers at Te Pūnaha Matatini and the University of Canterbury has developed a powerful new method to understand and address gender pay gaps in New Zealand’s public research sector – just as the country’s Crown Research Institutes face major restructuring.
Despite decades of awareness and campaigning, the latest figures from Stats NZ show an 8.2% national gender pay gap across Aotearoa. But while public service organisations are required to report pay gaps, current methods often fail to explain why they exist – or how to fix them.
This new research, published in the New Zealand Science Review, introduces a novel statistical approach that uses simulated data and causal modelling to pinpoint the drivers of pay inequity. The method allows organisations to test the likely impact of specific interventions – such as promotions or salary adjustments – before implementing them.
The study focuses on Crown Research Institutes – government-owned research organisations that are currently being merged into three new entities. The authors argue this moment of change is a critical opportunity to embed equity into the foundations of the new system.
“Crown Research Institutes have both the data and the expertise to lead the way on pay equity,” says lead author Dr Tom Moore. “We’re hoping this method can form a focal point for collaboration between human resources teams and data scientists as the new Public Research Organisations form.”
“Crown Research Institutes have both the data and expertise to lead the way on pay equity.” – lead author Dr Tom Moore.
The researchers used simulated salary-band data to reflect the structure of a typical Crown Research Institute, accounting for factors like role type, tenure, and full-time status. They found that simplistic interventions – like giving all women a flat pay increase – often fail to address deeper structural issues. In contrast, targeted changes informed by causal analysis can significantly reduce disparities.
“We wanted to move beyond just identifying pay gaps to actually testing what works to close them,” says co-author Dr Camilla Penney. “Our approach helps organisations understand the complex factors behind pay disparities and design smarter, more effective solutions.”
The study also highlights the importance of collaboration between human resources teams and data scientists, and calls for greater attention to intersectional pay gaps affecting Māori, Pasifika, and gender-diverse staff.
“Pay equity is not just a numbers game – real people’s livelihoods are at stake. So it’s about fairness, transparency, and making sure our public institutions reflect the values we hold as a country,” says Dr Penney.
“Pay equity is not just a numbers game – real people’s livelihoods are at stake.” – Dr Camilla Penney
This study builds upon previous work by Te Pūnaha Matatini researchers that has tested whether men and women are paid different wages for adding the same amount of value to their employer, shown that women are disproportionately under-represented in senior academic positions within New Zealand universities, and that under-representation of women in science increases with rising status within the hierarchy.
Towards effective interventions to address gender pay gaps: A causal approach in simulated salary band data – New Zealand Science Review

Disrupting the currents causing health inequality
29 May 2025
A collaboration between complex systems researcher Anna Matheson and illustrator Hanna Breurkes. Edited by Anna Brown.
Imagine you’re watching a river flow through a beautiful valley. At first glance, the river seems peaceful, carrying water gently downstream. But if you look closely, you’ll notice the current shaping everything it touches — carving paths, altering landscapes, and influencing every living community it flows through.
The reality of health inequality is much like this river — it isn’t just an outcome you measure at a single point in time, but an ongoing process constantly shaping people’s lives. Just like a river’s path is determined by terrain, rocks, and weather, health inequality is created and sustained by complex societal factors — economic policies, historical injustices, environmental conditions, and unequal power dynamics.
Picture a family living near the banks of this metaphorical river, stuck in overcrowded housing, juggling multiple low-paying jobs, struggling to access nutritious food and quality healthcare. These conditions don’t exist in isolation; they intertwine and reinforce one another, pulling the family deeper into hardship, much like powerful currents pulling everything in their path downstream.
The evidence for this dynamic is clear. Studies around the world show a social gradient in health: the lower you sit on the socioeconomic ladder, the poorer your health outcomes tend to be. Consider the famous Whitehall studies from the UK, where even civil servants with stable employment showed significantly worse health if they occupied lower-ranking jobs. Their limited control over work and life created constant stress, wearing down their health just as relentless currents erode the riverbank.
In New Zealand, this pattern is starkly evident, with people in the poorest neighbourhoods living about a decade less than those in the richest areas. Globally, too, this pattern of inequality is profound — life expectancy in materially poorer countries is roughly 33 years shorter than those with high incomes.
The data shows us a consistent pattern. But its regularity masks the twists and turns and constant movement of the people and artefacts it represents. It also masks the ease and strength of water that flows downwards. But rivers begin their existence at the height of mountains, where the action of water vapour has defied gravity to rise and help fill and refill trickles and torrents. A slower, dispersed and generative journey upwards.
Traditional solutions to health inequalities often resemble building small dams or bridges, addressing more immediate and singular issues like encouraging healthier eating or increasing health screenings. While valuable, these measures seldom alter the source of the river’s underlying current.
To change this flow, it is helpful to understand that our measurements of health inequality are simplifications, removed from their rich and constantly moving context. This reality is a complex interconnected web of ecosystems where changes in one area have potential to ripple outward, altering future outcomes. For inequality, ‘power’ is the rising vapour that can connect the downstream with the source of the flow upstream. And for health, the power-to-act is most impactful when people closest to the issues possess it.
Imagine local communities as expert guides intimately familiar with their unique stretch of the river. Even with plentiful information and evidence, they still need their rich local insights and lived experiences, as only they know exactly where currents are strongest and how to navigate hidden obstacles. When communities hold genuine power, they can steer resources and interventions to protect and nurture the environments and social conditions critical to their wellbeing, creating the foundations to withstand sparse or turbulent waters.
Yet the influence of empowered communities extends beyond their immediate surroundings. Local norms, practices, and innovations that serve community needs can send ripples upstream and vapour skywards — reshaping distant systems that perpetuate health inequalities. Just as water evaporates and rises, only to replenish mountain streams, the grassroots actions of communities can ascend, influencing policies and where resources are put, that in turn can remake relationships of trust and power. Local knowledge and actions become powerful currents of change, capable of even transforming economic and governance systems.
Health inequality is about more than a pattern of outcomes; it is the result of a dynamic and ongoing process, continuously shaping and reshaping the circumstances in which people live — across generations. To truly alter the river’s course, solutions must leave communities with the power to act collectively, not only to navigate the currents but to rise and influence the sources upstream themselves. Engaging with the connected flow of real relationships is where there is potential for disruption that can lead to system change.
Instead of cycles of inequality, we can foster cycles of regeneration, creating healthier and more resilient futures for everyone along the river’s path.
Anna Matheson is a principal investigator with Te Pūnaha Matatini who leads the research project Ki te toi o te ora: System change to reverse health inequality and environmental degradation.
Hanna Breurkes is a designer and illustrator who is passionate about designing to improve wellbeing and is inspired by nature.
Anna Brown is a principal investigator with Te Pūnaha Matatini who believes that design has a role to play in delivering public good.

The view beyond your valley
5 May 2025
A collaboration between researchers Richard Arnold, April Boland, Zoë Brown and Rebecca Priestley and illustrator Hanna Breurkes. Edited by Jonathan Burgess.
It’s 2025, and in Aotearoa New Zealand there are a growing number of people who believe that the Earth is flat.
That’s an extraordinary statement, coming from a technologically advanced society living more than 2,000 years after Greek philosopher Eratosthenes showed the Earth was round. Most Greek scholars of his time believed that the Earth was a sphere – they observed that ships’ hulls disappeared over the horizon before their masts, and saw the curved shadow of the Earth on the Moon during lunar eclipses – but Eratosthenes proved the Earth’s surface was curved and determined its size. To do this, he made observations of the lengths of shadows cast by the sun at two points a known distance apart on the river Nile and used simple mathematics to demonstrate the circumference of the globe Earth.
Observation is the most fundamental scientific activity and all human beings are observers – it’s how we make sense of our lives and the world. So why, in a country where scientists have been found to be very highly trusted, do some people stop trusting the science derived from observations?
Some people distrust scientific consensus if it challenges their own observations. Our 2021 interview with the mayor of Westland showed he relied only on his own observations, rather than the advice of the international climate science community, in judging whether or not the climate was changing. “Every year I’ve taken a photo on the first of May off my veranda at home,” he said. “Every year, the snow arrives two or three days before, or on the day, and it hasn’t changed in 20 years. I don’t think I’ve had a year that it hasn’t been the same. Some years, you know, a bit more snow than others, but they’re observations that I make personally.” [1]
Our interviews with a small community of high country sheep farmers showed they know their land well, and are expert observers of the weather and interpreters of weather forecasts. They have to be, their livelihoods depend on it. But they mistakenly lump weather forecasts and climate projections together. Because national weather forecasts can’t accurately forecast the weather in their own valleys very far ahead, they distrusted projections for future climate, asking whether “anyone is qualified to work that out”?
Similarly, people we interviewed who believed in a flat Earth relied on their own local observations – for example, that large bodies of water look flat – and they rejected the geometrical proof of curvature by Eratosthenes, or photographs of the globe Earth taken from space, or even the nature of gravity. In one of a series of interviews with flat Earth believers, the interviewee said, “the water’s surface is flat, it will always find its level. So, to me, it makes a lot more sense that [the Earth is] a ‘flat’ thing, than a ball spinning 1600 kilometers around at the circumference, not throwing any water off anywhere, because there’s this other magical force all pulling it inwards at the same time [gravity].” [2]
We’re very good at observing our surroundings – we each know our own valley very well. The trouble is, we can’t easily see the next valley, or the wider landscape. But science sees more than we can see as individuals. Because it’s an activity carried out by thousands of people, science can see far beyond our individual valleys, it can draw conclusions based on far more data than one individual could ever collect, and can see changes over periods longer than single lifetimes.
Like the ancient parable of the blind men and the elephant – in which the man who feels the trunk of the elephant believes he has encountered a giant snake, and the man who encounters a leg says the elephant is a pillar like a tree-trunk, and so on – we can find out so much more about the world if we work collectively than if we only rely on individual observations.
Some flat Earth believers told us that their disbelief in a round Earth was motivated by religion, but for others it came from a conviction that there is a widespread conspiracy to deceive and control people. They felt so alienated from science that they questioned some fundamental principles that we take for granted, such as gravity. For others a distrust of climate science is motivated by a dislike of policies that follow from accepting it; not everyone wants to swap their car for a bicycle or change to a plant-based diet. And some farmers don’t want to have to introduce what they see as costly changes to farming practices.
How did we get here? The method that Eratosthenes used for calculating the circumference of the Earth over two thousand years ago is still taught in schools, as is the chemistry of the greenhouse effect. Most of the farmers we spoke to had university qualifications – they knew about science. And we’ve interviewed flat Earth believers with strong instincts about the scientific method, about careful testing and experimenting.
So good science education and communication aren’t enough on their own.
When a stranger comes to your valley with news of the outside world, trust is not automatic – especially if they’re bringing bad news, or telling you to change your life. As scientists, we are often seen as strangers. So before we focus too much on communicating the science that we think is important, we need to start building trust with different communities living in different valleys. Because the challenges that we face are bigger than our own valleys.
[1] Westland mayor Bruce Smith, quoted in Rebecca Priestley, End Times (Te Herenga Waka University Press, Wellington, 2023), p.140.
[2] April Boland, ‘Don’t push me, I’m close to the edge: Flat Earth adherence in Aotearoa New Zealand’, PhD thesis (in progress), School of Science in Society, Te Herenga Waka Victoria University of Wellington.
Richard Arnold, April Boland, Zoë Brown and Rebecca Priestley are exploring how individuals and communities in Aotearoa New Zealand engage with, and trust (or mis-trust), science and scientists as part of the Science, statistics and the media project at Te Pūnaha Matatini.
Hanna Breurkes is a designer and illustrator who works with Toi Āria: Design for Public Good. She is based in Te Whanganui-a-Tara. You can see more of her work at hannabreurkes.nz.

The forest of discovery: Government research organisations in Aotearoa New Zealand
23 April 2025
A collaboration between science system researcher Brittany Bennenbroek and illustrator Jean Donaldson. Edited by Jonathan Burgess.
Deep in the heart of Aotearoa, a mighty kauri forest once stood. These ancient trees represented the pursuit of knowledge, their towering trunks standing firm against the winds of uncertainty.
They were first planted in 1926 when the government recognised that a more coordinated approach to research was essential for national development. Those who tended this land believed in cultivating strong, enduring trees that would stand the test of time, their knowledge and resources serving future generations.
This was the era of the Department of Scientific and Industrial Research (DSIR), which was established to coordinate and support scientific research. Over the decades, the DSIR grew into a powerhouse of discovery, employing thousands of scientists across fields like climate science, geology, marine research, and agriculture. It played a crucial role in wartime innovation and later provided research that supported industries and environmental management.
The DSIR was carefully cultivated, with scientists given increasing autonomy, allowing deep-rooted knowledge to flourish. Over time, these trees grew strong, their canopy stretching wide, sheltering diverse fields of research.
As the years passed by, the forest changed hands many times, with each new group of owners bringing with them their own vision and priorities, transforming the landscape of research funding.
In the late 1980s, the political winds shifted dramatically. The DSIR was instructed to adopt a “user-pays” approach to research, and before long, all research funding was made contestable. This meant that trees could no longer be planted for their potential; they would need to compete for space and resources, and every tree would need to prove its worth.
The old, slow-growing trees, those that might one day yield the most profound discoveries, were no longer seen as a priority. The shift marked a move away from collective stewardship toward a more transactional model, where steady growth and deep roots gave way to efficiency and return on investment.
In 1992, the government restructured the system entirely. The DSIR was disestablished, and the land cleared for a new kind of tree. Fast-growing and commercially valuable, pine trees promised quick returns, aligning with an era driven by immediate economic benefits. The Crown Research Institutes (CRIs) were established, dividing the forest into zones aligned with key industries like agriculture, forestry, and environmental science.
Unlike the DSIR, which was largely government-funded, the CRIs were designed as government-owned companies and operated under a contestable funding model that aimed to encourage competition, accountability, and commercialisation. As a result, they often prioritised projects that had immediate practical applications. It marked a significant shift – from long-term discovery-led research to a fragmented system driven by shorter-term economic priorities.
As time passed, the limitations of the pine forest became apparent. While fast to grow, these trees were shallow-rooted and vulnerable, especially when storms rolled in. Unlike old-growth forests that absorb impact and offer protection, the pine forest proved far less resilient. A forest optimised for quick economic returns may look productive, but it can leave the landscape exposed when conditions change.
Seeking to bridge the fragmented system and bring greater collaboration to the research landscape, in 2014, the government established ten National Science Challenges. These were designed to address Aotearoa’s most significant science-related issues and opportunities, and unite researchers across CRIs, universities and other organisations. Through these partnerships, new shoots began to regrow a more connected forest, linking people, knowledge, and purpose.
In 2024, the National Science Challenges came to an end, and the forest became restless. Now, in 2025, things have shifted again. The pine trees are being cleared, ready for a new kind of forest to take root: the Public Research Organisations (PROs).
We stand at a crossroads, and what this forest may become is still uncertain. We have an opportunity to grow a rich and resilient ecosystem – diverse by design, rooted in care, curiosity, and collaboration. A forest shaped by partnership across disciplines, institutions, and communities, capable of bearing different kinds of fruit. One where long-term sustainability is prioritised alongside innovation, and where the seeds we sow today will support future generations, withstand the storms to come, and lead to research that delivers lasting, real-world impact.
Brittany Bennenbroek completed an internship with Te Pūnaha Matatini’s Kindness in Science project as part of her Master of Science in Society study at Te Herenga Waka – Victoria University of Wellington.
Jean Donaldson is a designer and illustrator who works with Toi Āria: Design for Public Good. She is based in Te Whanganui-a-Tara. You can see more of her work at https://jeanmanudesign.com/.

Yes, data can produce better policy – but it’s no substitute for real-world experience
7 April 2025
Anna Matheson, Te Herenga Waka — Victoria University of Wellington
Governments like to boast that “data-driven” policies are the best way to make fair, efficient decisions. They collect statistics, set targets and adjust strategies to suit.
But while data can be useful, it’s not neutral. There are biases and blind spots in the systems that produce the data. Worse, data often lacks the depth, context and responsiveness needed to drive real-world change.
The real questions are about who decides which data matter, how it’s interpreted – and what the change based on the data might look like.
Take the Social Investment Agency, for example. One of New Zealand’s best-known data-driven initiatives, it was established to improve the efficiency of social services using data and predictive analytics to identify individuals and families most at risk, directing funding accordingly.
The model is intended to guide early interventions and prevent long-term harm. And on paper, this appears to be a smart, targeted strategy. Yet it has also faced criticism over the risk of data-driven policies reducing individuals to measurable statistics, stripping away the complexity of lived experiences.
The result is that decision making remains centralised within government agencies rather than being shaped by the communities most affected.
What data can’t tell us
The Social Investment Agency also relies on Stats NZ’s Integrated Data Infrastructure, a database of anonymised administrative information. While a rich source for longitudinal research and policy development, this too has limitations.
It relies heavily on government-collected data, which may embed systemic bias and fail to represent communities accurately. Without accounting for context, some populations may be underrepresented or misrepresented, leading to skewed insights and misguided policy recommendations.
This kind of data is completely separate from the lived reality of the people the data describes. Māori in particular have been concerned about a lack community ownership and that the Integrated Data Infrastructure does not currently align with their own data sovereignty aspirations.
Given this greater likelihood of misrepresentation, Māori and Pasifika communities worry that data-driven funding models, on their own, fail to account for more holistic, whānau-centered approaches.
For instance, a predictive algorithm might flag a child as “at risk” based on socioeconomic indicators. But it would fail to also measure protective factors such as strong cultural connections, intergenerational knowledge and community leadership.
This is where the kaupapa Māori initiative Whānau Ora provides an alternative model. Instead of viewing individuals in isolation, it prioritises the needs of families to provide tailored housing, education, health and employment support.
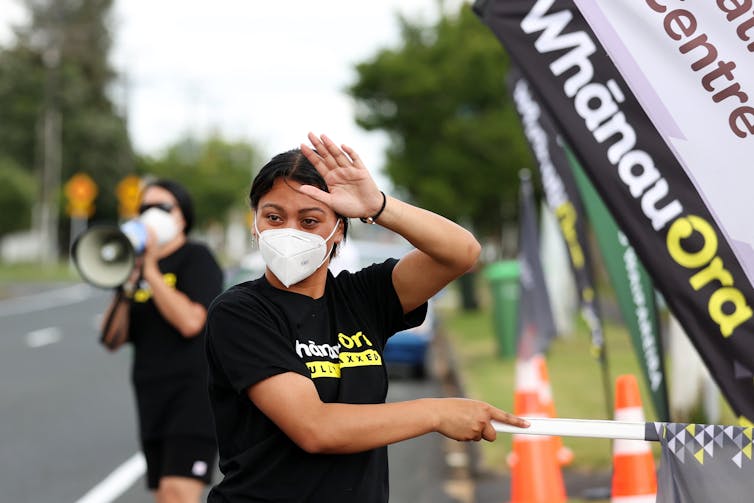
Image: A Whānau Ora COVID vaccination campaign in 2021 funded Māori health providers to reach at-risk communities in the North Island. Getty Images.
Change from the ground up
Funded by Te Puni Kōkiri/Ministry of Māori Development, Whānau Ora has been criticised in the past for the lack of measurable outputs data-driven systems can offer. But research has also shown community-led models produce better long-term outcomes than traditional, top-down, data-driven welfare and service delivery models.
A 2018 review found Whānau Ora strengthened family resilience, improved employment outcomes and increased educational engagement – for example, through supporting whānau into their own businesses and off social assistance.
Whānau Ora’s work strengthening community networks and building self-determination migh be harder to measure using standard metrics, but it has long-term economic and social benefits.
Similarly, data-driven approaches to disease prevention can fall short. While governments might rely on obesity rates or physical activity levels to shape interventions, these blunt measurements fail to capture the deeper social and economic factors that affect health.
Too often, strategies target individual behaviours – calorie counting, exercise tracking – assuming better data leads to better choices. But we know local conditions, including what financial and community resources are available, matter much more.
An example of this in action is Health New Zealand/Te Whatu Ora’s Healthy Families NZ division. With teams in ten communities around the country, it works to create local change to improve health.
Instead of simply telling people to eat better and exercise more, it has supported community action to reshape local environments so healthier choices become easier to make.
In South Auckland, for example, Healthy Families NZ has worked with local businesses to improve access to fresh, affordable food. In Invercargill, it has helped transform urban planning policies to expand green spaces for physical activity.
Data in perspective
Such initiatives recognise health is about more than just individuals. It is a shared outcome that results from systemic processes. Data-driven approaches by themselves struggle to capture these less measurable pathways and relationships.
That is not to say government-led, data-driven methods don’t often diagnose the problem correctly – just that they frequently fail to provide solutions that empower communities to make lasting change.
Rather than over-relying on data analytics to dictate funding, or on national health targets to guide the system, cross-sector and place-based initiatives such as Whānau Ora and Healthy Families NZ can teach us a lot about what works in the real world.
Data will always have an important role to play in shaping policy, but this requires a broader perspective. Data offers a tool for communities, not a substitute for their leadership and voice. Real system change happens when we fundamentally rethink how change happens, and who leads that change in the first place.
Anna Matheson, Principal Investigator, Te Pūnaha Matatini and Associate Professor in Public Health and Policy, Te Herenga Waka — Victoria University of Wellington
This article is republished from The Conversation under a Creative Commons license. Read the original article.

Your friends probably have more friends than you do
10 March 2025
A collaboration between network scientist Dion O’Neale, modeller and analyst Emily Harvey, and illustrator Hanna Breurkes. Edited by Jonathan Burgess.
One day in 1967, strange letters began arriving in letterboxes in Omaha, Nebraska. The letters had the name of a stockbroker in Boston, Massachusetts and instructions to send them on to a friend or acquaintance who might get the letter one step closer to that stockbroker.
This was part of a series of experiments by sociologist Stanley Milgram to explore how connected America was. Each time an envelope was passed on, the name of the new participant was recorded. Milgram found that it took only an average of six transfers between people for the message to navigate its way across the country between two complete strangers.
This finding gave us the well-known concept that a random pair of people in the world are separated by only six degrees of separation.
We now know that social networks have interesting structural features that are different from people being simply connected at random. These structural features have consequences for how things might spread on the network, whether that be information – like fake news or hilarious cat gifs – or communicable disease such as COVID-19 or HIV.
One of the many interesting structural features in social networks is known as a “heavy-tailed degree distribution”. Degree is network scientist speak for the number of connections that a node in the network has. In this case, each node is a person, and the degree is the number of friends each person has in a social network.
Facebook is a very countable example of a “friendship” network with explicit connections. The degree distribution is when you count up the number of people in a network who each have a certain number of connections. You might find that in a particular network ten people each have three connections, six people have four connections, five people have nine connections, and so on – until you reach that one person who seems to know almost everybody.
When we put the numbers from this degree distribution on a chart we find that the chart continues far out to the right – with a small but significant number of people having a large number of connections – we say that the degree distribution is heavy-tailed. These charts end up looking a little bit like the back half of a brontosaurus.
If a social network has a heavy-tailed degree distribution, it will contain some people who are very highly connected. When something spreading on the network arrives at one of these people, they have a large number of potential people to pass it on to. Highly connected people link up parts of the network that would otherwise be far apart.
The potential for highly connected individuals to spread things showed up in Milgram’s letter passing experiments. In one case, 24 of the letters that made their way from Omaha to Boston were passed to the target person at his home address, with 16 of those coming from the same preceding person. And over half of the letters that reached the target at his office came from only two other people in the preceding hop. The letters had found their way to the same small number of highly connected individuals who were then able to pass them on to their intended location in a single step.
The fact that most people have a connection to someone highly connected is almost an inevitable consequence of how social networks are connected. This is related to the friendship paradox, which says that your friends probably have more friends than you do.
The maths to prove that this is a general feature of most different types of networks can get a bit complicated, but it’s true enough in general that we can use the consequences of the friendship paradox in practical ways to monitor or influence things that might be spreading on a network.
Let’s say you want to detect whether the outbreak of some disease is coming. You could do this by simply selecting people at random, monitoring when they get sick, and watching to see when cases start to climb rapidly. A better choice would be to select some highly-connected individuals and monitor their health, since their larger number of connections means that they are likely to be infected sooner.
We usually don’t know in advance who these highly connected people are going to be. This is where things like the friendship paradox can help us. If we start with a randomly selected set of people and ask them each to name a friend then – on average – those friends are going to be more highly connected than the set of people that we started with. Even without knowing the entire structure of a network, we can use ideas like the friendship paradox to make useful guesses about the local connections around a specific point in the network.
And this isn’t something that’s just true in theory. Network scientists showed in a study involving an influenza outbreak at Harvard College that by picking students at random and then asking those students to name a friend, they were able to detect an influenza outbreak almost two weeks early by monitoring the set of friends instead of the randomly selected individuals.
We can also use this property of network structure to reduce the spread of disease, by targeting highly-connected people for interventions like vaccination. Doing so can slow the spread of disease by meaning that transmission pathways have to be much longer to reach people, giving more time to vaccinate, or otherwise protect others.
When you’re making decisions about something that spreads – whether it’s disease, ideas, money or electricity – considering the structure of the network that it will spread on is important for understanding the outcomes of that spreading process and can help you to make a decision with the best possible information.
Although they are everywhere in our lives, our intuition can fail us when it comes to thinking about networks. Remember – your friends probably have more friends than you do.
Read more about how something spreads across a network
Dion O’Neale co-leads the spreading processes on (multilayer and multiplex) networks project at Te Pūnaha Matatini.
Emily Harvey is a researcher on the spreading processes on (multilayer and multiplex) networks project at Te Pūnaha Matatini.
Hanna Breurkes is a designer and illustrator who is passionate about designing to improve wellbeing and is inspired by nature.

PhD scholarship on measuring the impact of lake health interventions
Image: Te Roto o Wairewa by Billie Ihaka.
4 March 2025
Applications are invited for a PhD scholarship to study the health of shallow coastal lakes in Aotearoa, currently in a state of decline driven by environmental factors and human activity.
A number of interventions have been trialled to improve the health of shallow coastal lakes with mixed success. A lack of rigorous monitoring data has meant that assessing the impact of these intervention fails to be proactive, responsive and effective. A better understanding of lake health is required to identify appropriate natural solutions or human interventions to reverse these declines.
Te Roto o Wairewa, a shallow polymictic shoreline lake on the southern side of Te Pātaka o Rākaihautū, Banks Peninsula, has had a troubled history. The catchment was deforested and the lake closed off to the sea in the 1860s and since that time the water has remained turbid and prone to toxic algal blooms. The customary and highly productive tuna (eel) fishery was on the verge of collapse due to the highly degraded state of the lake. Efforts by mana whenua (Wairewa Rūnanga – local Ngāi Tahu Māori community) have been instrumental in improving lake water quality, but further improvements are desired to ensure the well-being of both tuna and whānau (families).
You will work alongside another PhD student exploring mathematical models of spatio-temporal early warning signals. This component of the project aims to establish a rapid on-site lake water surveillance system to detect the fluctuations in key algal indicators (diatoms and cyanobacteria). This will involve a combination of conventional microscopy, molecular approaches and machine learning. The generated data will be used to inform the spatio-temporal warning system. The main aim is to assess the efficacy of small (e.g. sediment traps or floating vegetation mats) and large scale (e.g. Te Kōawa Ika o Wairewa, a large-scale fish pass system being constructed within the period of study) lake water interventions.
Eligibility
This scholarship is open to anyone who can be in New Zealand and meets the requirements to enrol in a PhD at the relevant institution. We are happy to consider students from a diverse range of fields including ecology, biology, the geosciences or similar quantitative disciplines. Interested students should have a growing background in hydrological monitoring and associated numerical methods (working with R or Python), with at least some background in molecular techniques (e.g. genomic DNA).
The project will also involve significant interaction with groups invested in the health of the lake, including mana whenua and environmental agencies. To this effect, one of the outcomes of the broader project is the construction of a digital twin of the lake as a communication tool, so experience in gaming development (e.g. Unreal Engine) will be an advantage. The successful candidate will hold, or expect to complete soon, an honours or masters level qualification, with a significant research project.
Applicants from all countries and backgrounds are actively encouraged to apply. Members of underrepresented groups are very welcome, as are students with families. Our research group aims to achieve work-life balance within a productive scientific environment.
Location
You will be based at the University of Canterbury and will work with Dr Matiu Prebble and Professor Graham Donovan. You will work alongside the team at the Waterways Centre for Freshwater Management.
You will also be part of Te Pūnaha Matatini, the Aotearoa New Zealand Centre of Research Excellence for Complex Systems. Te Pūnaha Matatini brings together ‘many faces’ – different disciplines, ways of thought, methods, and crucially, people – to define, and then solve, society’s thorny interconnected problems. Te Pūnaha Matatini has an active whānau group which supports early career researchers, committed to the Te Pūnaha Matatini values of manaakitanga and whakawhanaungatanga, offering supportive tuakana / teina learning environments.
Contact
If you have any questions, please contact Matiu Prebble at matiu.prebble@canterbury.ac.nz.
Financial details
- Full tuition fees
- Stipend of NZ$35,000 per year (tax free)
Start date
The start date is flexible, but would preferably be in the first half of 2025.
How to apply
Send an email expressing your interest, along with a CV, academic record, and list of three potential referees to matiu.prebble@canterbury.ac.nz.
Due date
Applications will be considered until the position is filled.

Balancing with Antarctica
17 February 2025
A collaboration between PhD candidate Kristin Wilson and illustrator Jean Donaldson. Edited by Jonathan Burgess.
If you travel south from Aotearoa New Zealand, you will eventually hit the landmass known today as Antarctica.
Antarctica wasn’t always there, at the bottom of the Earth. Over one billion years ago it was in the Northern Hemisphere, hanging out with India, Australia, and Laurentia (early North America). And before that? Well, we can’t trace back that far. But we do know that Antarctica’s oldest rocks are around 3.9 billion years old, meaning Antarctica has been around for most of Earth’s long life.
By 30 million years ago, Antarctica had travelled south and was isolated from all other landmasses, locked over the South Pole. Cooling and freezing followed, creating challenging conditions for Antarctica’s land-based life forms. Trees and vertebrate animals disappeared. Much smaller life forms such as springtails, mites, roundworms, and tardigrades, learned to adapt to the freezing temperatures, lack of running water, and seasons of extreme sunlight and darkness.
More than 99% of Antarctica is now covered by ice. In the ice-free locations, the ancient continent of gravel and rock is still visible. The Dry Valleys – near Ross Island – are the largest ice-free region (~4,500 km2). These deep U-shaped valleys hint at fjords long emptied.
It was not until the 1950s that Antarctica became part of the modern human world, in the lead up to the International Geophysical Year – a global scientific effort undertaken during 1957 and 1958. Research bases were built. Scientific programmes were devised and implemented. Charles Keeling began measuring monthly CO2 concentrations in the atmosphere.
After the success of the International Geophysical Year, the twelve nations who had conducted research in Antarctica agreed to continue doing science there, to stop trying to agree who owned which part, and formalised this agreement in the 1959 Antarctic Treaty.
Today, Antarctica is one of Earth’s global commons. Like the high seas, the atmosphere, and outer space, Antarctica isn’t owned by any single sovereign state. The Antarctic Treaty has expanded to include 57 nations (as of 2024), of which 29 nations, who do “substantial research activity” in Antarctica make decisions annually.
Antarctica is governed in the “interests of mankind.” Yes, that includes you.
The stated environmental principles for governance include: peace, science, environmental, ecological, wilderness, aesthetic, and intrinsic values. These values are guides to support the overall goal of “the comprehensive protection of the Antarctic environment and dependent and associated ecosystems.”
Antarctica is a fragile and unique place, so there is cause for concern. In 1996, Warwick Vincent asked a difficult question, “can the scientific value of research activities be clearly justified against unavoidable impacts?”
The need to balance between different environmental principles in Antarctica and the importance of scientific knowledge to humankind is a reoccurring goal since – at least – the adoption of the Protocol on Environmental Protection to the Antarctic Treaty in 1991.
What does ‘balance’ mean in reality?
In 1990, Elinor Ostrom cautioned against “relying on metaphors as the foundation for policy advice” as they lead to “results substantially different from those presumed to be likely.”
Complexity science, the field which studies complex phenomena, is interested in real-world puzzles like these.
From the perspective of complex systems, a balance (or an imbalance), is a description of the relationship between two or more things. In mathematical terms, either arithmetic or geometric, it refers to an equality.
Human preoccupations with determining, obtaining, and maintaining equalities appear in many concerns of life – health, economics, law, ethics, spirituality – and can be traced in history through ancient Egypt, classical Greece, the Middle Ages, to today. In Aotearoa New Zealand we recognise equality in the story of Goldilocks and the concept of utu.
In Antarctica, arithmetic logic is found in the linking of scientific and environmental values as scientific gains outweighing environmental impacts, formalised in an impact assessment process structured by increasing levels of environmental impact.
Complex systems are also dynamic systems, so balance takes on an additional meaning, referring to the stability (or instability) of a system overall. Understanding the processes of stability often takes centre stage, articulated through regulation, feedback loops, and emergence.
But what does balance mean in reality for Antarctica? We know that scientific knowledge is important to humankind, but every human activity there has an impact on its environment and ecosystems.
How do we balance Antarctica’s environmental importance to the planet with the importance of scientific knowledge to humankind?
Kristin Wilson has been exploring balance in Antarctica as a PhD candidate on Te Pūnaha Matatini’s Human activity in the McMurdo Dry Valleys – Rescue, knowledge and understanding our role as a vector of change project.
Jean Donaldson is a designer and illustrator who works with Toi Āria: Design for Public Good. She is based in Te Whanganui-a-Tara. You can see more of her work at https://jeanmanudesign.com/.

The geography of health inequities
A collaboration between health geographer Jesse Whitehead and illustrator Hanna Breurkes. Edited by Jonathan Burgess.
22 January 2025
“Dad, when are you going to die?”
I slowly open my groggy eyes to see my six-year-old daughter staring at me with a look of concern on her face. It’s 6am on a Monday morning, and I’m not ready for this.
But it is kind of my job. And not just as a parent who is supposed to somehow have an answer to all life’s impossible questions.
As a demographer and health geographer, it’s my job to think about how environments impact our health. I like maps, and use data to understand more about people and place – and how environments impact our health.
We can’t live forever, and none of us know when we’ll die. But some of us will live longer, healthier lives than others.
In Aotearoa New Zealand, health inequities are stark, persistent, and avoidable. These inequities, defined as unfair and preventable differences in health outcomes, challenge our perception of Aotearoa as a fair society. The social determinants of health – factors like housing, employment, education, and access to health services – play a significant role in these disparities.
For instance, the average life expectancy for pākeha men in Aotearoa is about 81 years, while for Māori men, it’s 73 years. This gap has widened since 2014. People living in the poorest areas of Aotearoa have a life expectancy nine years lower than those in wealthier areas.
A 1998 report from the National Health Committee clearly linked poverty to ill health, noting that the financially worst-off have the highest rates of illness and early death. Despite the passage of time, these findings remain relevant. Recent data reveals that young people under 30 living in remote areas are up to three times more likely to die than their urban counterparts.
We only found this out in 2023. What’s changed in rural Aotearoa? It turns out that health outcomes haven’t changed, but the way we look at data about people and place has. Historically, definitions of rurality in Aotearoa grouped small towns together with large urban centres based on their urban form – basically having streets, footpaths and suburbs – and lifestyle blocks on the edges of cities were considered rural. This led to misleading health research outcomes.
Under the leadership of Professor Gary Nixon, we developed a new classification system for rurality that better reflects health realities. This new system revealed that previous classifications underestimated rural health outcomes, particularly for preventable deaths.
Definitions were masking inequalities.
The conditions in which we are born, grow, work, live, and age create health differences. Rural populations in New Zealand tend to be older and poorer, with lower incomes, fewer formal qualifications, and less access to technology. These conditions are worse for Māori, especially in remote areas where 73% live in high-deprivation zones.
Poor access to health services further exacerbates these disparities. Rural communities consistently highlight access as a key issue, and the government aims to improve this through its rural health strategy.
The Covid-19 pandemic provided a stark example of how access to services impacts health outcomes. During the vaccine rollout, urban areas had numerous vaccination centres, while rural areas were often left with few options.
In the most vaccinated suburbs in Auckland, Wellington and Queenstown, the longest drive time to a vaccination centre was just five minutes. In Murupara, labelled the nations “slowest town”, the median age was 29 years – meaning that people had only just become eligible for the vaccine – and the nearest clinic was almost an hour’s drive away.
This led to significant disparities in vaccination rates, highlighting the need for a more equitable approach to health service delivery.
Looking ahead, Aotearoa faces significant demographic changes. By the 2050s, one quarter of the population could be over 65, with the number of people over 85 doubling. This ageing population will stretch health resources, with older individuals already occupying a significant portion of hospital beds. At the same time, the Māori population is young and growing, with a median age of just 27. Balancing the needs of these two demographic groups will be a major challenge.
Climate change adds another layer of complexity, bringing new health challenges such as extreme heat and more frequent severe weather events. Cyclone Gabrielle, for example, caused $14 billion in damage and significant disruption to health services. Building resilient and equitable health systems that can withstand these challenges is crucial.
Addressing health inequities in New Zealand requires a multifaceted approach. We need to improve living conditions, ensure fair access to health services, and prepare for future demographic and environmental changes.
These are complicated, intersecting and interrelated issues that nobody has a simple solution to. But research can play a part. If we can better understand the complex nuance of these problems, we can work together to create better solutions.
“Daaaadd! When are you going to die!??”
Ahh, that’s right – it’s Monday morning. How do I explain all that to a six year old?
“Well darling, nobody knows exactly when they’ll die. But if we work together to create a fairer society, we can all live longer healthier lives.”
Jesse Whitehead is principal investigator with Te Pūnaha Matatini, who focuses on impact and equity through health geography and demography.
Hanna is a designer and illustrator who is passionate about designing to improve wellbeing and is inspired by nature.